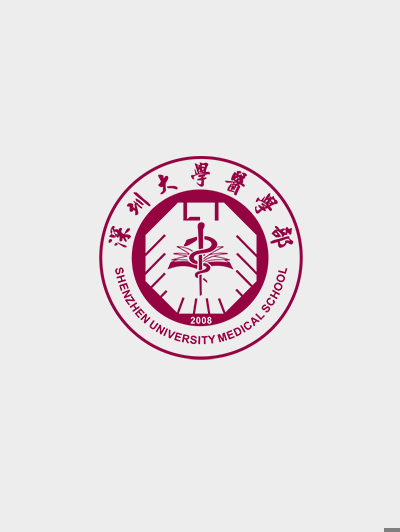
Wang, Yejun (Assistant Professor) Assistant Professor
Basic Medical School
Assistant Professor
Department of Cell Biology and Medical Genetics
BIOGRAPHICAL SKETCH
NAME: Wang, Yejun
POSITION TITLE: Assistant Professor of Bioinformatics, Shenzhen University Health Science Center
EDUCATION/TRAINING
INSTITUTION AND LOCATION |
DEGREE
|
Completion Date MM/YYYY
|
FIELD OF STUDY
|
---|---|---|---|
Peking University Health Science Center, Beijing, China |
B. Med |
07/2004 |
Clinical Medicine |
Peking University Health Science Center, Beijing, China |
M.Med |
07/2007 |
Pathogenic Biology |
The Chinese University of Hong Kong, Hong Kong |
Ph.D. |
07/2011 |
Bioinformatics |
The Chinese University of Hong Kong, Hong Kong |
Postdoctoral |
08/2012 |
Bioinformatics |
University of Saskatchewan, SK, CA
|
Postdoctoral
|
11/2014
|
Infectious Diseases; Bioinformatics |
A. Personal Statement
My research interests include: (1) bacterial systems biology, and (2) bioinformatics and its application in human health studies(especially in the Chinese population). I founded the first bioinformatics virtual lab in Shenzhen University Health Science Center, HCD laboratory ( http://www.szu-bioinf.org ), combining cloud platforms and machine learning techniques, specifically deep learning, to resolve the haplotypes, multi-clones and metagenomes of disease-associated genomes or pathogens. During the first 3 years that the HCD lab was initiated, we developed ~30 bioinformtic software tools, including seven for which national software copyright s have been registered . We developed the first hybrid de novo assembly and annotation system for large, complex genomes, and the first genetic risk evaluation system for pre - eclampsia in Chinese women. I was invited to edit a bioinformatic protocol book published by Springer. My lab has published 16 research papers in peer-reviewed professional journals and four book chapters in the past two years.
B. Positions and Honors
Positions and Employment
11/2014 – present Assistant Professor, Department of Cell Biology & Genetics, Shenzhen University Health Science Center, Shenzhen, China
Other activities
Book editor: Transcritptome Data Analysis, Springer, 2018/4/3
Peer reviewer for the journals: Bioinformatics, Briefings in Bioinformatics, BMC Bioinformatics, BMC Genomics, Applied and Environmental Microbiology, Journal of Bacteriology, Frontiers in Microbiology
C. Contributions to Science (*: corresponding/co-corresponding author)
A list of my representative publications in past 5 years is enclosed at the end of this document.
1. Bacterial systems biology
Life is so complicated that even a free-living bacterial cell with a small genome is far beyond what we have understood. My research team is dedicated to understanding the maintenance and evolving mechanisms of the simplest independent life system, the evolution of bacterial genomes, virulence systems and individual genes, and bacterial population surviving strategies, using integrated resources and computational techniques. Simply, we investigate bacteria in four dimensions: genes, sub-networks, genomes/cells and communities. To better annotate the FUN (Function_UNkown) genes, we use both forward and reverse annotation strategies. For example, we forwardly observed the gene evolution and the driving force, proposed the maximum-parsimony mutation theory for the first time and found structure-constrained mutation pressure in LRR proteins (Infect Immun, 2016). We also developed the most popular and accurate bacterial virulence effector predictors to help reversely annotate bacterial gene function (Bioinformatics, 2011; BMC Genomics, 2014). At the sub-network level, as an example, we systematically investigated the distribution and evolution of bacterial type III secretion systems (T3SSs), their effectors and transcript regulation circuits, and for the first time clustered the T3SSs into 13 groups, identified >8,000 new effectors, and found low-level conservation and high-level variation of T3SS transcriptional regulation (Environ Microbiol, 2017). At the genome/cell level, we re-constructed the ancient genome of Salmonella and dynamically illustrated the evolution traces of Salmonella genomes (Hu et al, in preparation). For bacterial communities, we have been focusing on biofilms and probiotics. As one of the major participants and the only bioinformatic researcher, I studied with Prof. Aaron P. White and Dr. Keith D. MacKenzie (University of Saskatchewan, CA), and reported for the first time the bet-hedging evolutionary strategies of Salmonella, developing to aggregates for environmental survival and to planktonic cells for invasion (Infect Immun, 2015; selected by editors as the spotlight).
1) Hu Y, Huang H, Hui X, Cheng X, White AP, Zhao Z*, Wang Y*. (2016). Distribution and Evolution of Yersinia Leucine-Rich Repeat Proteins. Infect Immun. 84(8):2243-54.
2) Wang Y, Zhang Q, Sun MA, Guo D*. (2011). High-accuracy prediction of bacterial type III secreted effectors based on position-specific amino acid composition profiles. Bioinformatics. 27(6):777-84
3) Wang Y*, Wei X, Bao H, Liu SL*. (2014). Prediction of bacterial type IV secreted effectors by C-terminal features. BMC Genomics. 15:50.
4) Hu Y, Huang H, Cheng X, Shu X, White AP, Stavrinides J, Köster W, Zhu G, Zhao Z, Wang Y*. (2017). A global survey of bacterial type III secretion systems and their effectors. Environ Microbiol. 19(10):3879-3895.
5) MacKenzie KD, Wang Y, Shivak DJ, Wong CS, Hoffman LJ, Lam S, Kröger C, Cameron AD, Townsend HG, Köster W, White AP*. (2015). Bistable expression of CsgD in Salmonella enterica serovar Typhimurium connects virulence to persistence. Infect Immun. 83(6):2312-26. (selected by editors as spotlight)
2. Disease-genotype association in Chinese population
Our achievements in technology are amazing. However, we and our relatives are still suffering aging and health problems every day. My research group aims to contribute to understanding the common health issues of the population. To do so, we are developing various computational tools to assist analyzing genomes and haplotypes, identify associations between different diseases and genotypes, and develop models to help monitor the risks or early onset of different diseases, and guide prognosis and treatment selection. Specifically, we are developing methods to improve statistical power in capturing significant biomarkers or other features (Bioinformatics, 2017; J Cancer, 2017). Tools are also under development for better analysis of the haplotype genome or transcriptome of human parasites (BMC Genomics, 2015; J Pathol, 2018). Genome variations are being detected and compared between various diseased and healthy Chinese people, and advanced machine learning models are being developed to facilitate the application of the findings.
1) Hui X, Hu Y, Sun MA, Shu X, Han R, Ge Q, Wang Y*. (2017). EBT: a statistic test identifying moderate size of significant features with balanced power and precision for genome-wide rate comparisons. Bioinformatics. 33(17):2631-2641.
2) Zhang S, Xu Y, Hui X, Yang F, Hu Y, Shao J, Liang H*, Wang Y*. (2017). Improvement in prediction of prostate cancer prognosis with somatic mutational signatures. J Cancer. 8(16):3261-3267.
3) Wang Y, MacKenzie KD, White AP*. (2015). An empirical strategy to detect bacterial transcript structure from directional RNA-seq transcriptome data. BMC Genomics. 16:359.
4) Shu XS*, Zhao Y, Sun Y, Zhong L, Cheng Y, Zhang Y, Ning K, Tao Q, Wang Y*, Ying Y*. (2018). The epigenetic modifier PBRM1 restricts the basal activity of the innate immune system by repressing retinoic acid-inducible gene-I-like receptor signalling and is a potential prognostic biomarker for colon cancer. J Pathol. 244(1):36-48.
D. Research Support (ongoing)
Shenzhen Science and Technology Innovation Commission grant (JCYJ20160307115221141) 01/01/2017 to 12/31/2018
Yejun Wang, PI ¥300,000
Translocation mechanism of a Salmonella type III secreted effector SteA
Shenzhen overseas peacock research fund 01/01/2016 to 12/31/2018
Yejun Wang, PI ¥3,000,000
High-accurate haplotyping a Chinese-Han human genome and its applications in cancer research
E. Publications
Representative journal publications in past 5 years (since 2013) (*: corresponding author)
1. Shu XS*, Zhao Y, Sun Y, Zhong L, Cheng Y, Zhang Y, Ning K, Tao Q, Wang Y*, Ying Y*. (2018). The epigenetic modifier PBRM1 restricts the basal activity of the innate immune system by repressing retinoic acid-inducible gene-I-like receptor signalling and is a potential prognostic biomarker for colon cancer. J Pathol. 244(1):36-48.
2. Zhang S1, Xu Y1, Hui X, Yang F, Hu Y, Shao J, Liang H*, Wang Y*. (2017). Improvement in prediction of prostate cancer prognosis with somatic mutational signatures. J Cancer, 8(16):3261-3267.
3. Guo Z1, Cheng X1, Hui X, Shu X, White AP, Hu Y, Wang Y*. (2017). Prediction of New Bacterial Type III Secreted Effectors with a Recursive Hidden Markov Model Profile-Alignment Strategy. Current Bioinformatics, DOI: 10.2174/1574893612666170725122633.
4. Zhang Q, Fan X, Wang Y, Sun MA, Shao J, Guo D*. (2017). BPP: a sequence-based algorithm for branch point prediction. Bioinformatics, 33(20):3166-3172.
5. Hui X, Hu Y, Sun MA, Shu X, Han R, Ge Q, Wang Y*. (2017). EBT: a statistic test identifying moderate size of significant features with balanced power and precision for genome-wide rate comparisons. Bioinformatics, 33(17):2631-2641.
6. Hu Y, Huang H, Cheng X, Shu X, White AP, Stavrinides J, Köster W, Zhu G, Zhao Z, Wang Y*. (2017). A global survey of bacterial type III secretion systems and their effectors. Environ Microbiol, 19(10):3879-3895.
7. Sun MA, Wang Y, Zhang Q, Xia Y, Ge W, Guo D*. (2017). Prediction of reversible disulfide based on features from local structural signatures. BMC Genomics, 18(1):279.
8. Wang J1, Wang Y1, Gao C, Jiang L, Guo D*. (2017). PPero, a Computational Model for Plant PTS1 Type Peroxisomal Protein Prediction. PLoS One, 12(1):e0168912.
9. Han R, Huang G, Wang Y, Xu Y, Hu Y, Jiang W, Wang T*, Xiao T*, Zheng D*. (2016). Increased gene expression noise in human cancers is correlated with low p53 and immune activities as well as late stage cancer. Oncotarget, 7(44):72011-72020.
10. Sun MA, Zhang Q, Wang Y, Ge W, Guo D*. (2016). Prediction of redox-sensitive cysteines using sequential distance and other sequence-based features. BMC Bioinformatics, 17(1):316.
11. Shivak DJ, MacKenzie KD, Watson NL, Pasternak JA, Jones BD, Wang Y, DeVinney R, Wilson HL, Surette MG, White AP*. (2016). A Modular, Tn7-Based System for Making Bioluminescent or Fluorescent Salmonella and Escherichia coli Strains. Appl Environ Microbiol, 82(16):4931-43.
12. Zhou L, Cheng L, He Y, Gu Y, Wang Y, Wang C*. (2016). Association of gene polymorphisms of FV, FII, MTHFR, SERPINE1, CTLA4, IL10, and TNFalpha with pre-eclampsia in Chinese women. Inflamm Res, 65(9):717-24.
13. Hu Y1, Huang H1, Hui X, Cheng X, White AP, Zhao Z*, Wang Y*. (2016). Distribution and Evolution of Yersinia Leucine-Rich Repeat Proteins. Infect Immun, 84(8):2243-54.
14. Madampage CA*, Rawlyk N, Crockford G, Wang Y, White AP, Brownlie R, Van Donkersgoed J, Dorin C, Potter A. (2015). Reverse vaccinology as an approach for developing Histophilus somni vaccine candidates. Biologicals, 43(6):444-51.
15. Perez-Casal J*, Prysliak T, Maina T, Wang Y, Townsend H, Berverov E, Nkando I, Wesonga H, Liljander A, Jores J, Naessens J, Gerdts V, Potter A. (2015). Analysis of immune responses to recombinant proteins from strains of Mycoplasma mycoides subsp. mycoides, the causative agent of contagious bovine pleuropneumonia. Vet Immunol Immunopathol, 168(1-2):103-10.
16. Wang Y, MacKenzie KD, White AP*. (2015). An empirical strategy to detect bacterial transcript structure from directional RNA-seq transcriptome data. BMC Genomics, 16:359.
17. MacKenzie KD, Wang Y, Shivak DJ, Wong CS, Hoffman LJL, Lam S, Kröger C, Cameron ADS, Townsend HGG, Köster W, White AP. (2015). Bistable Expression of CsgD in Salmonella Connects Virulence to Persistence. Infection and immunity, 83(6):2312-26.
18. Gao C, Cai Y, Wang Y, Kang BH, Aniento F, Robinson DG, Jiang L*. (2014). Retention mechanisms for ER and Golgi membrane proteins. Trends Plant Sci, 19(8):508-515.
19. Wang Y1*, Wei X1, Bao H, Liu SL*. (2014). Prediction of bacterial type IV secreted effectors by C-terminal features. BMC Genomics, 15:50.
20. Li C1, Shao J1, Wang Y1, Li W1, Guo D, Yan B, Xia Y*, Peng M*. (2013). Analysis of banana transcriptome and global gene expression profiles in banana roots in response to infection by Race 1 and Race 4 of Fusarium oxysporum f. sp. Cubense. BMC Genomics, 14:851.
21. Wang Y, Sun M, Bao H, Zhang Q, Guo D*. (2013). Effective identification of bacterial type III secretion signals using joint element features. PLoS ONE, 8(4):e59754.
22. Zou QH, Li RQ, Wang YJ, Liu SL*. (2013). Identification of genes to differentiate closely related Salmonella lineages. PLoS ONE, 8(2):e55988.
23. Wang Y*, Sun M, Bao H, White AP*. (2013). T3_MM: a Markov model effectively classifies bacterial type III secretion signals. PLoS ONE, 8(3):e58173. *Co-corresponding authors.
Book and Book Chapters
1. Wang Y, Sun MA (Eds). Transcriptome Data Analysis: Methods and Protocols. Methods in Molecular Biology Vol. 1751, Springer, Humana Press, 2018. ISSN: 1064-3745. DOI: 10.1007/978-1-4939-7710-9. (Book)
2. Sun MA, Zhai R, Zhang Q, Wang Y. (2018). Identification and Expression Analysis of Long Intergenic Noncoding RNAs. Methods Mol Biol. 1751:139-152. (Chapter)
3. Wang Y, Sun MA, White AP. (2018). RNA-Seq-Based Transcript Structure Analysis with TrBorderExt. Methods Mol Biol. 1751:89-99. (Chapter)
4. Sun MA, Shao X, Wang Y. (2018). Microarray Data Analysis for Transcriptome Profiling. Methods Mol Biol. 1751:17-33. (Chapter)
5. Cheng H, Wang Y, Sun MA. (2018). Comparison of Gene Expression Profiles in Nonmodel Eukaryotic Organisms with RNA-Seq. Methods Mol Biol.1751:3-16. (Chapter)
用户登录
还没有账号?
立即注册