Deciphering p53 dynamics and cell fate in DNA damage response using mathematical modeling
Review Article
Nanfei Yang, Tingzhe Sun & Pingping Shen
Genome Instability & Disease 1,265–277(2020)
Abstract
The tumor suppressor p53 is activated in response to cellular stresses. The transcription factor p53 can activate the expression of numerous genes leading to cell cycle arrest, senescence or apoptosis. The p53 network exhibits complex stimulus-dependent dynamics under stressed and non-stressed conditions. Mathematical models contribute significantly to enhanced understanding of p53 network topology. In this review, we discuss the evolution of kinetic p53 modeling, multiple mechanisms for distinct p53 dynamics, and how the temporal p53 dynamics determine cell fate over the last 2 decades. The Information encoding and decoding strategies through p53 signaling network enable cells to undergo appropriate cellular outcomes.
Introduction
The mammalian cells are constantly confronted with intrinsic stress (Lowndes 2001). Genomic rearrangement and replication may result in DNA lesions (So et al. 2017). Cellular metabolism can generate reactive oxygen species to extensively attack biomolecules (Kultz 2005). Environmental stressors such as irradiation also jeopardize the integrity of genome. The multicellular organism should initiate a program to halt cell division and prevent propagation of mutations in the event of excessive DNA damage. The DNA damage will initiate a signaling cascade known as DNA damage response (DDR) (Shikazono et al. 2009). The DDR may promote DNA repair and limit normal cell cycle progression until damages are fully resolved. The critical role of DDR is highlighted by the identification of various cancer-associated genes within DNA repair signaling (Krenning et al. 2019). A notable mediator in DDR is the ‘guardian of the genome’ p53 and TP53 loss usually leads to defects in DNA repair and cancer predisposition (Tang et al. 2020; Lane 1992). The DNA double strand breaks (DSBs), which are classified as the clustered type of severe DNA lesions, can induce rapid trans-autophosphorylation of ataxia-telangiectasia mutated protein (ATM) (Bakkenist and Kastan 2003), which then phosphorylates p53 along with other substrates (Banin et al. 1998). ATM-mediated p53 phosphorylation activates p53 and initiates a series of tightly controlled downstream responses (Fig. 1a). The ultraviolet (UV) light, however, activates ATM and Rad3-related (ATR) to relay damaging signals to p53 via phosphorylation (Tibbetts et al. 1999).
Fig. 1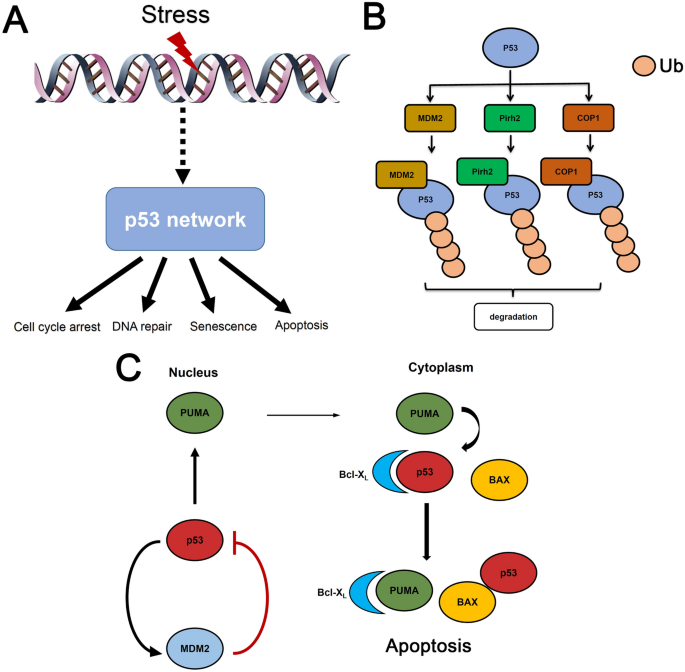
P53 is a central node in cellular stress responses. a The p53 protein is activated by multiple types of stimuli such as ironizing radiation, UV light, oncogenic stress. P53 encodes the upstream signals from intrinsic or extrinsic stresses into distinct dynamics and elicits diverse cellular outcomes (e.g. DNA damage repair, apoptosis, cell cycle arrest or senescence). b Redundant role of MDM2, Pirh2 and COP1. p53 can induce MDM2, Pirh2 and COP1 expression and these proteins in turn target p53 for proteasome-mediated degradation. c The coupled nuclear and cytoplasmic functions of p53. In nuclear, p53 exerts its tumor suppressive function as a transcription factor. Stress-induced de-repression of p53 may lead to elevated p53 in both nucleus and cytoplasm. P53-induced PUMA can neutralize the anti-apoptotic Bcl-xL proteins and release cytoplasmic p53-associated with Bcl-xL. The cytoplasmic p53 further activates pro-apoptotic Bax and induces apoptosis
Full size imageThe cellular p53 abundance is tightly regulated by negative feedback loops. A distinguished negative feedback loop relies upon p53 induced mdm2 transcription and MDM2 protein can in turn promote p53 degradation (Wu et al. 1993). In addition, p53 induces the expression of a phosphatase Wip1, and Wip1 can deactivate ATM to restrict p53 activation (Batchelor et al. 2008). The subsets of p53 downstream transcriptional targets reflect a stimulus dependency (Hafner et al. 2017; Batchelor and Loewer 2017; Batchelor et al. 2011). To enable intricate control, the p53 signaling exhibits versatile dynamics in response to DNA damage. Early work implied that p53 performed damped oscillation (damped oscillation indicates that the heights are vanishing following consecutive pulses) when cells were challenged with γ-irradiation (Lev Bar-Or et al. 2000; Hu et al. 2007). Later studies in individual cells demonstrated that p53 was accumulated in sustained pulses with relatively concise periods (Lahav et al. 2004; Geva-Zatorsky et al. 2006; Ramalingam et al. 2007). The information of stimulus strength (e.g. irradiation doses or number of DSBs) may be encoded in the number of p53 pulses and the level of p53 downstream targets (Harton et al. 2019). The qualitative oscillatory p53 dynamics is highly conserved in cell lines from other species (e.g. monkey and rat), although the duration seems to be variable (Stewart-Ornstein et al. 2017). Even under normally physiological conditions, the intrinsic DNA damage can also trigger spontaneous p53 pulses with regular pulse morphology (Loewer et al. 2010).
In addition to γ-irradiation, topoisomerase inhibitors such as etoposide (Eto) and neocarzinostatin (NCS) also result in continuously recurring DSBs but lead to distinct p53 dynamics other than periodic pulses (Chen et al. 2013; Yang et al. 2018). The p53 dynamics may shift from uniform pulses to monotonic increases and significantly elevated p53 abundance will initiate apoptosis (Chen et al. 2013; Yang et al. 2018). As a result, distinct p53 dynamics lead to different cellular behaviors and targeted perturbations may alter cell fates (Purvis et al. 2012).
Given the extensive repertoire of biomolecules involved in p53 interaction, using conventional approaches alone may prevent an intuitive understanding of p53 network (Sun and Cui 2015). Computational strategies can provide a more comprehensive view about the network topology. A mathematical model also helps unravel novel hypotheses regarding the emergent behaviors in p53 signaling network. In current review, we focused on mechanistic p53 models and how versatile p53 dynamics influence cell fates in DNA damage response.
Dynamic constraints in p53 model construction
Most studies have focused on unraveling the interactions within p53 network in DDR. P53 is kept at very low levels when cells are under non-stressed conditions (Muller and Vousden 2013). Some notable negative regulators contribute to p53 attenuation in the absence of stresses (Brooks and Gu 2006). These functionally redundant ubiquitin ligases include MDM2, Pirh2, and COP1, which all target p53 for proteasome-mediated degradation (Brooks and Gu 2006) (Fig. 1b). The binding between p53 and MDM2 plays a central role of in mathematical p53 models due to their mutual interactions in vivo (Fig. 2a) (Jones et al. 1995). The negative feedback loop between p53 and MDM2 has prompted several early models which show oscillatory p53 patterns in response to DSBs (Lev Bar-Or et al. 2000; Tiana et al. 2002; Monk 2003). These models, however, showed damped p53 oscillations at population levels.
Fig. 2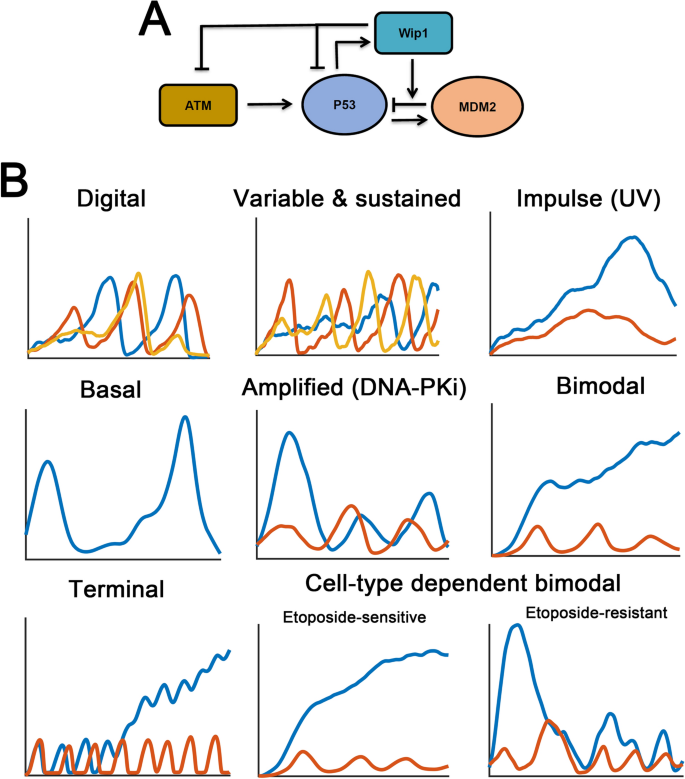
Stimulus- and cell-type dependent p53 dynamics. a The core negative feedback loop in p53 signaling (p53–MDM2 and ATM–p53–Wip1 negative regulatory loops). The Wip1 phosphatase can also dephosphorylate p53 and MDM2, respectively. Arrows indicate activation, the blunt arrows represent deactivation. b The diverse p53 dynamic patterns show a stimulus- and cell-type dependency. Basal p53 pulses are observed under non-stressed conditions (see text for details). In ‘digital’ scenario, one to three p53 pulses are shown in response to identical DNA damage. In the ‘variable & sustained’ case, three stochastic trajectories are shown. Two temporal p53 dynamics reveal variability in pulsing characteristics (red and yellow). The left case indicates only random fluctuation without a detectable pitch (blue). In ‘basal’ scenario, spontaneous p53 pulses are unraveled with long inter-spike duration. For the remain scenarios, higher (blue) or lower (red) stimuli or damaging agents are used to show the diversity in p53 dynamics
Full size imageObservations at single cell resolution indicated that p53 performs digital or sustained pulses (Lahav et al. 2004; Geva-Zatorsky et al. 2006) suggesting that the modeling framework should be modified. Several methods are used to generate digital (Fig. 2b, ‘Digital’) or undamped p53 oscillations (undamped oscillation indicates that the amplitudes are maintained, Fig. 2b, ‘Variable & sustained’). An intuitive modification is introduction of explicit delays in the model. The negative feedback alone can only produce damped pulses. However, if an explicit delay is presented (i.e. in the form of delay differential equations), the system has the potential to show undamped pulses (Tyson et al. 2003). A p53-MDM2 negative feedback with time delays can maintain the sustainable dynamics of p53 pulses (Tyson et al. 2003; Tiana et al. 2002; Bottani and Grammaticos 2007). However, large explicit time delays used for maintaining undamped p53 oscillations are not always feasible in normal physiologically settings. An alternative approach was to increase the time delays by additional intermediates in p53 signaling network. A notable strategy is incorporating ATM-mediated p53 phosphorylation and accelerated MDM2 degradation (Ma et al. 2005). The number of unrepaired DSBs controls the duration in which the p53 system is localized within limit cycle regions through a Hopf bifurcation (Tyson 2006). As a result, higher irradiation may ignite more p53 pulses whereas low doses of γ-rays only allow a few pulses (Wagner et al. 2005). Similar modeling framework was also used in a model by Tyson and colleagues (Ciliberto et al. 2005). These pioneering models have provided critical insight into the ‘digital’ p53 pulses. When the fluorescence signals were recorded for longer duration, Alon and colleagues found that p53 indeed exhibited sustained pulses with concise periods and variable amplitudes (Geva-Zatorsky et al. 2006). Distinguished phenomenological models were also proposed to investigate the general architectures responsible for undamped p53 pulses and their models faithfully recapitulate the experimental observations at individual cells (Geva-Zatorsky et al. 2006; Abou-Jaoude et al. 2011; Proctor and Gray 2008; Cai and Yuan 2009). Some models also indicated that noises in p53 production rates serve as the major source for heterogeneous p53 responses (Geva-Zatorsky et al. 2006). Furthermore, a relaxation oscillator was designated for reproducing sustained p53 pulses. A relaxation oscillator is well characterized in dynamical systems theory (Batchelor and Loewer 2017). A relaxation oscillator features positive feedback loops. As previously described, p53 network is pervaded with positive feedbacks, such as PTEN/Akt, ISG15, p53 autoactivation or microRNA (miRNA)-mediated effects (Harris and Levine 2005; Moore et al. 2015; Sun et al. 2010; Gao et al. 2020; Park et al. 2016; Wee et al. 2009; Puszynski et al. 2008). The coupled negative–positive feedback regulation, therefore, creates a relaxation oscillator to sustain the undamped p53 pulses, although the absence of specific positive feedback loops in certain cell lines challenges the coordinated role of these positive feedbacks (Batchelor and Loewer 2017). Positive feedback loop may also occur upstream of p53 protein. The existence of ATM switch by MRN or MDC1 complex has been extensively explored (Mouri et al. 2009; Sun et al. 2009). Switch-like ATM activation in response to DSBs upstream of p53 was experimentally verified and guarantees undamped p53 pulses in a recent work (Monke et al. 2017).
A further ‘constraint’ in p53 modeling argues that the ATM–p53–Wip1 negative feedback loop should be incorporated especially in DDR (Fig. 2a). The requirement of additional negative feedback loops is theoretically postulated via Fourier analysis in which the oscillatory p53 pattern is better approximated by a linear third-order model (Geva-Zatorsky et al. 2010). Specifically, the role of Wip1 is not restricted to ATM dephosphorylation. Wip1 can also dephosphorylate phosphorylated p53 and MDM2 (Shimada and Nakanishi 2013). In a recent model, Stewart-Ornstein et al. used a simplified p53 model without p53–Wip1 feedback loop and identified that DNA repair efficiency and the ATM activity (Stewart-Ornstein and Lahav 2017). Therefore, we argue that in p53 models without DNA damage, it seems that the ATM–p53–Wip1 loops is not a prerequisite for an ‘accurate’ p53 model, whereas the alternative p53–Wip1–MDM2 negative feedback loop might be considered. We recently discussed the role of p53–Wip1–MDM2 negative feedback loop during cancer progression (Sun and Mu 2020) and the results showed that it may provide robustness to p53 signaling. Therefore, incorporating additional feedback loops other than p53–Wip1–ATM and p53–MDM2 negative feedback loops may provide emerging features to p53 dynamic responses.
Dynamic properties of p53 signaling
Excitability of p53 dynamics
Early mathematical p53 models demonstrated that recurrent p53 patterns can be ascribed to limit cycle or harmonic oscillations (Jolma et al. 2010; Tyson 2006). Limit cycles or harmonic oscillations suggest that the p53 dynamics operates in an autonomous manner. However, later experimental observations showed that p53 pulses may exhibit properties of excitability (Batchelor et al. 2008). Even under non-stressed conditions, spontaneous p53 pulses can be triggered by intrinsic DNA damage from normal cell cycle progression (Fig. 2b, ‘Basal’) (Loewer et al. 2010). As a result, the network structures should be constrained with novel features. An excitable system suggests that a p53 pulse will be initiated once the stimulus (DSBs) or activated ATM level is above a certain threshold (Loewer et al. 2010). The excitable properties of p53 signaling relies on the checkpoint kinase ATM, and treatment with ATM inhibitors will undoubtedly abolish the basal p53 pulses (Loewer et al. 2010). Several mathematical models have been constructed to describe the spontaneous p53 pulses (Chong et al. 2015; Sun et al. 2011). Chong et al. explain the spontaneous nature via a deterministic model (Chong et al. 2015), our own model extensively considers the intrinsic DNA damage during DNA replication (single strand lesions converted into DSBs) and chromosome de-condensation induced DSBs in mitosis (Su 2006; Vilenchik and Knudson 2003; Ichijima et al. 2005; Sun et al. 2011). The intrinsic DSBs are randomly interspersed when DNA replicates itself, and triggers spontaneous pulses via excitable mechanisms. Our model also quantitatively recapitulates the major experimental findings in Loewer et al.’s work (Loewer et al. 2010). A notation is that in Bottani et al.’s model, ‘non-stressed condition’ is also mentioned and p53 performs sustained pulses under ‘non-stressed condition’ (Bottani and Grammaticos 2007). However, ‘non-stressed condition’ in Bottani et al.’s model corresponds to the state without DNA damage, which is in stark contrast to intrinsic DSBs in Loewer et al.’s experiments (2010).
The excitable p53 pulses are highly dependent on the stimuli. Therefore, measurements of the DSB dynamics at single cell resolution will be helpful. Using fluorescent reporters, Loewer et al. found that the initial number of DSBs and repair are highly variable. A linear relation between DSBs and the p53 dynamics was observed, in which higher number of DSBs induces more p53 pulses (Loewer et al. 2013). We constructed a mechanistic model with only canonical p53 negative feedback loops (p53–MDM2 and p53–Wip1–ATM) (Wu et al. 2016). We found that owing to the excitable nature of p53 pulses, increasing the number of DSBs indeed ignites more p53 pulses and our simulation quantitatively matched the experimental data (Wu et al. 2016; Loewer et al. 2013). Recent evidence revealed that the DSB repair kinetics vary substantially across isogenic cell population (Karanam et al. 2012; Monke et al. 2017). Simple and complex DSBs coexist with different repairing efficiencies and kinetics (Monke et al. 2017). These novel features have been incorporated into a new p53 model (Monke et al. 2017). The stochasticity in DSB repair, ATM activation switch and two canonical negative feedback loops were combined. This model has unraveled novel features in which Wip1 protein levels affect the possibility of triggering p53 pulses providing a possible way to regulate the excitability in p53 signaling (Monke et al. 2017). The complex excitability of p53 pulses is further potentiated by a recent work. Finzel et al. found that under DNA-PK inhibitor treatments, the first p53 pulses under DSBs are substantially amplified. This is primarily induced by DNA-PK-mediated ATM inhibition and their prediction was verified later in experiments (Fig. 2b, ‘Amplified DNA-PKi’) (Finzel et al. 2016; Zhou et al. 2017). We modeled this effect in silico by incorporating the interactions among ATM, ATR and DNA-PK upstream of p53. We identified that DNA-PK-mediated ATM inhibition is indispensable for generating the enlarged first p53 pulses. The information flow is accumulated to the first p53 pulses under DNA-PK inhibition (Sun et al. 2017). In addition, most studies emphasize a negative role of MDM2 in p53 regulation, MDM2 can individually or corroborate with MDMX to positively regulate p53 synthesis (Malbert-Colas et al. 2014; Gajjar et al. 2012). As a result, the excitability of p53 pulses is revisited with novel positive regulation of p53 by MDM2. Elias showed that the dual regulatory role of MDM2 upon p53 can extensively reproduce the excitable p53 pulses in silico (Elias 2017). Kim et al. modeled the role of p53–RORα positive feedback loop and showed that this loop can maintain the sustainability of p53 pulses (Kim and Jackson 2013). By refining the parameters, the positive regulatory effect of MDM2 on p53 induction is indispensable for bimodal p53 responses (Sun and Cui 2014). Collectively, the recurrent p53 dynamics now has shifted from autonomous oscillations to excitable pulses.
Stimulus dependence of p53 dynamics
Pulsatile p53 dynamics has been previously regarded as the ‘golden rule’ especially in DDR. Mathematical models with non-pulsing p53 patterns have seldom been postulated. Since p53 pulses highly rely on the ATM–p53–Wip1 loop in DDR (Batchelor et al. 2008), it seems possible if the ATM–p53–Wip1 negative loop is silenced for example, under ultraviolet (UV) irradiation (Unsal-Kacmaz et al. 2002). UV primarily induces DNA crosslinkers and signals through ATR and Checkpoint kinase 1 (Chk1) (Lecona and Fernandez-Capetillo 2018; Saldivar et al. 2017). As a result, the ATM–p53–Wip1 loop will be bypassed even in cells with intact ATM pathway. Therefore, we proposed a two-component minimal model with interlocked positive and negative feedback loops (Sun et al. 2010). We argued that if the strength of negative feedback is strongly attenuated, positive feedback regulation may play a dominant role in DDR (Sun et al. 2010). In the event of significantly weakened negative feedbacks (e.g. UV irradiation), the p53 dynamics may potentially behave as a monotonic increasing pattern or bistable switch (Sun et al. 2010; Wang et al. 2018). This counterintuitive idea of non-pulsing p53 in DDR seems impossible. However, a prominent work later by Batchelor et al. indeed showed that p53 pulses cannot been observed in cells challenged by UV primarily due to ATM–Chk2–p53 bypass (Fig. 2b, ‘Impulse UV’) (Batchelor et al. 2011). The amplitude and duration of p53 dynamics are increased with the strength of UV irradiation (Batchelor et al. 2011). Their experimental data, therefore, support our model predictions (Sun et al. 2010). Hunziker et al. extended this issue by a simple p53 model and showed that p53 exhibits stress-dependent behaviors in response to DNA damage, oncogenic stress or Hypoxia (Hunziker et al. 2010). Furthermore, Pu et al. also evaluated a non-pulsing bistable behavior of p53 by coupled nuclear and cytoplasmic p53 functions (Pu et al. 2010). The coupled nuclear and cytoplasmic function indicates that nuclear p53 induces the expression of MDM2, which targets p53 for degradation. Cellular stress disrupts this inhibition, allowing p53 accumulation both in the nucleus and cytoplasm. In cytoplasm, p53 is sequestered by anti-apoptotic Bcl-xL. PUMA, another downstream target of nuclear p53, can interrupt the Bcl-xL–p53 interaction. The released p53 can promote apoptosis by, for example, activating Bax (Fig. 1c) (Green and Kroemer 2009). However, the ATM–p53–Wip1 bypass is not mentioned in their work. The ‘lessons’ from non-pulsing p53, however, have prompted numerous novel studies.
The p53 dynamics in response to DNA damage reveals non-pulsatile patterns if p53-MDM2 interaction or ATM–p53–Wip1 negative feedback is interrupted (Choi et al. 2012). However, Choi et al.’s work mainly focused on the critical role of combinatorial Nutlin-3 treatment and wip1 depletion in transitions among cellular states. A pioneering study by Chen et al. challenged the pulsatile p53 dynamics in response to etoposide-induced DSBs (Chen et al. 2013). They examined the p53 dynamics after etoposide treatments in U-2 OS cells with a fluorescent p53 reporter. Surprisingly, they observed that p53 performs uniform pulses when etoposide doses are low. However, higher etoposide dosage will lead to monotonic increase in p53 levels and finally induce significant apoptosis (Fig. 2b, ‘Bimodal’) (Chen et al. 2013). We constructed the first mathematical model that recapitulates the bimodal p53 dynamics (Sun and Cui 2014). We incorporated MDM2-mediated p53 induction and found that the positive role of MDM2 on p53 translation outcompetes the effect of p53–MDM2 negative feedback and results in monotonic p53 elevation. Later, Chen et al. constructed an apoptotic p53 model to recapitulate their own experimental findings (Chen et al. 2014; Chen et al. 2013). Under higher doses of etoposide challenge, induction or stability of p53 proteins is enhanced, therefore, positive feedback in p53 signaling may be stronger than negative feedback and p53 levels will become monotonically increased (Chen et al. 2014; Sun and Cui 2014). The stimulus dependency in p53 dynamics are further evaluated in more models with detailed sensitivity analysis (Ma and Dai 2018, 2019).
The stimulus-dependent features of p53 has been revisited recently. Wu et al. have discovered distinct p53 dynamics under low or high doses of doxorubicin (Dox) treatments. They found that higher Dox dosage leads to sustained elevation of p53 in contrast to the p53 pulses under low-dose Dox treatment (Wu et al. 2017). We constructed a model to recapitulate this behavior. Our model showed that p53 dynamics was gradually shifted from typically sustained pulses to variable pulses with a terminal elevation when Dox doses were increased (Fig. 2b, ‘terminal’). Mechanistically, the effect of MDM2–p53 mRNA binding to enhance p53 mRNA translation (Gajjar et al. 2012) was considered in the model and steady accumulation of MDM2 under p53 pulses may instead increase the abundance of p53 mRNAs (Sun et al. 2018). Therefore, the positive feedback again outcompetes the negative feedback on p53 levels and p53 will exhibit a terminal elevation as the number of pulses increases. The effective p53 integral was not significantly altered with varying Dox doses. The biphasic p53 patterns could dictate cell fate in combination with binding affinity for p53 target genes (Sun et al. 2018). Yang et al. evaluated the cell-type-dependent p53 activation in etoposide-sensitive (U-2 OS, A375, A549) and -resistant cell lines (MCF7, 769-P, HepG2) (Yang et al. 2018). In etoposide-sensitive cells, p53 shows uniform pulses or monotonic increases depending on the stimulus strength as previously described (Yang et al. 2018; Chen et al. 2013). However, in etoposide-resistant cell lines, p53 shows extended large pulses when high dose etoposide was used. The bimodal p53 response depends upon coupled feedforward and feedback loops (ATM-MDM2 and ATM-p53-MDM2, respectively). As a result, Yang et al.’s work has advanced our knowledge about ‘bimodal’ p53 responses in different cell lines (Fig. 2b, ‘Cell-type dependent bimodal’). The bimodal p53 behaviors also point to a druggable mechanism in etoposide-resistant cells by modulating p53 dynamics (Yang et al. 2018). Various other DNA damaging agents (e.g. NCS) should be used to identify versatile p53 dynamics in resistant cells. Taken together, the balance between positive and negative feedback loops in p53 signaling indeed confers various p53 dynamics. Furthermore, the stimulus-dependent p53 dynamics may fulfill its role in information encoding leading to potential cell fate decision in DDR. The successful replication of these complex dynamics using mathematical models in DDR relies on the excitability of p53 pulses (Sun et al. 2017, 2018; Wu et al. 2016; Sun et al. 2011).
P53 dynamics in cell fate decision
Given the tumor suppressive role of p53 network against cancer progression, the mechanism for p53 mediated cell fate decision has been an active area of research. Ironizing radiation induces p53 pulses. The DNA damage is allowed to repair when p53 performs pulses and normal cell cycle is halted. Cell cycle reentry is authorized after DNA damage is low or fully resolved (Iwamoto et al. 2011). When p53 performs pulsatile dynamics, numerous effectors with diverse physiological functions are specifically or simultaneously induced. As a result, the choice to terminal fate is determined by the coordinated roles of these effectors. This accounts for most theoretical studies of p53 modeling.
An early p53 model demonstrates that DNA damage levels affect cell fate by incorporating cell cycle and apoptotic pathway (Bose and Ghosh 2007). Tyson and colleagues explored a series of modeling framework and faithfully replicates the digital p53 pulses (Fig. 3a) (Zhang et al. 2007). The total p53 abundance is classified int three functional different species: ‘p53 lurker’, ‘p53 helper’ and ‘p53 killer’. The ‘p53 killer’ activates apoptotic genes while ‘p53 helper’ dictates p21 induction and cell cycle arrest. The p53-induced p53DINP1 controls the conversion from ‘p53 helper’ to ‘p53 killer’ and, therefore, determines cell fate. If DNA damage is maintained at high levels, ‘p53 killer’ will be accumulated via p53 DINP1 feedback loop and trigger apoptosis. The intricate interplay among ‘p53 lurker’, ‘p53 helper’ and ‘p53 killer’ has provided a mechanism for p53-mediated cell fate decision (Zhang et al. 2007). The ‘p53 helper’ and ‘p53 killer’ method was further extended in a more sophisticated model for intrinsic apoptotic pathway (Zhang et al. 2009a). A combined experimental and theoretical work explored the multiple mechanisms in cell cycle arrest and found that distinct cell cycle mechanisms can act in concert to elicit reliable cell cycle control (Toettcher et al. 2009). Zhang et al. extended Tyson and colleagues’ model with refined DSB repair module (Fig. 3b) (Zhang et al. 2009b). They incorporated repressive effect from p53 on DNA damage repair in the model, although some studies have supported a positive role of p53 during DSB fixation (Serrano et al. 2013; Gao et al. 2000). If DNA damage persists, longer duration in limit cycle regions will lead to significantly more apoptotic gene expression and cells will undergo apoptotic cell death. We also proposed a simplified model which integrates ATM activation switch, p53 oscillator and downstream effector induction (e.g. p53 up-regulated modulator of apoptosis, PUMA) (Fig. 3c) (Sun et al. 2009). PUMA is a notable proapoptotic regulator which also couples nuclear and cytoplasmic p53 function (Nakano and Vousden 2001; Chipuk et al. 2005). Consequently, p53-induced PUMA expression may simultaneously initiate both intrinsic and extrinsic apoptotic pathways (Hengartner 2000). The coupled nuclear and cytoplasmic role of p53 is further studied in a more sophisticated model with significant match to experimental data (Liu et al. 2014). The interconversion of functional p53 species and transcriptional induction of downstream effectors when DNA damage is above the threshold coordinately dictate cell fate in theoretical models.
Fig. 3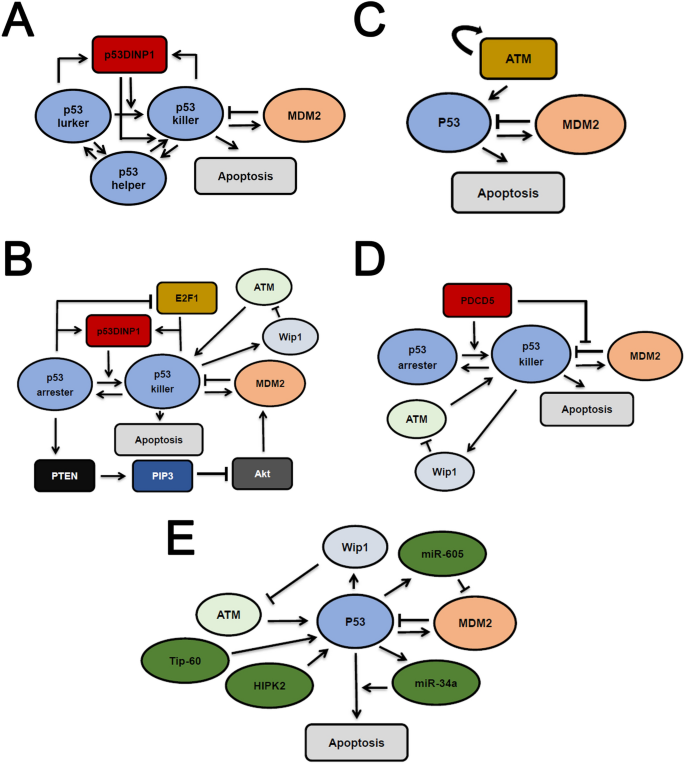
Multiple mechanisms in p53 models for cell fate decision. a A simplified representation of p53 models with three functionally different p53 forms (‘p53 helper’, ‘p53 lurker’, ‘p53 killer’). The p53DINP1 promotes the transition into ‘p53 killer’. b Multiple mechanisms responsible for p53 induced cell fate decision. PTEN/Akt, E2F1 pathways are incorporated individually in separate models. c A simply p53 model with cell fate decision, ATM autoactivation switch is integrated. p53-induced PUMA expression contributes to apoptosis induction. d PDCD5 activates p53 and deactivates MDM2, and this serves as the major mechanism for p53-mediated cell fate decision. e Multiple cofactors (miR-605, mR-34a, HIPK2 or Tip-60) function individually or in a combinatorial manner to determine cell fate. Arrows indicate activation, the blunt arrows represent deactivation. For simplicity, only essential interactions are shown in each panel. Note that in b–d, all putative interactions in several models are incorporated and represented altogether for simplicity
Full size imageSome new players are integrated into models to update the mode of p53-mediated cell fate decision. The coordination between E2F1 and p53 signaling has been investigated by Zhang et al. (2010) (Fig. 3b). E2F1 undergoes a transition from sustained pulses at low levels to monotonic elevation if the DSBs are not faithfully repaired. P53 also exhibits a phase transition from short to long duration pulses. As a result, p53 and E2F1 corporately induce cell cycle arrest with repairable DNA damage and promotes apoptosis when DSBs become overwhelming. The PTEN/Akt pathway is further considered by the same authors. Zhang et al. showed that incorporating PTEN/Akt signaling creates a novel two-phase p53 dynamics (Fig. 3b) (Zhang et al. 2011). P53 initially performs undamped pulses which corresponds to a survival state. When DNA damage level is high, p53 abundance will be rapidly elevated leading to cell apoptosis. The two models by the same authors used a general ‘two phase’ framework in which the phase transition results in a terminal fate (Zhang et al. 2010, 2011).
Programmed cell death 5 (PDCD5) is another apoptosis-associated molecule with broad impact upon intrinsic and extrinsic apoptotic pathways (Cheng et al. 2008; Xu et al. 2009). Zhuge et al. developed an integrated model to elucidate the central role of PDCD5 (Fig. 3d) (Zhuge et al. 2011). They noted that PDCD5-mediated Bax translocation to mitochondria determines the apoptotic switch in the DDR. DNA damage-induced p53 activation of p53-MDM2 negative feedback loop was integrated in their first model (Zhuge et al. 2011). Later, this model was revisited to incorporate the effect of PDCD5 on MDM2 (Zhuge et al. 2016). In their model, PDCD5 can inhibit MDM2 mediated p53 degradation and promotes MDM2 degradation, which is reminiscent of ATM activity in DDR (Cheng et al. 2009). Consequently, the cellular PDCD5 activity dramatically shapes p53 dynamics from low level fluctuation, sustained oscillation to high level induction (Zhuge et al. 2016). Notably, p53 also participates in intrinsic apoptotic pathway and functions as a ‘BH3-only’ protein (Hemann and Lowe 2006). The interplay between PDCD5 and p53 can synergistically regulate both intrinsic and extrinsic pathways to dictate cell fate.
Other p53 cofactors have been considered in mathematical models of cell fate decision. Generally, the levels of unrepaired DNA damage (DSBs) initiate different programs in p53 signaling pathway to discriminate survival and death. For example, sequential induction of Hzf or ASPP reliably triggers cell cycle arrest or apoptosis, depending on the extent of DNA damage (Zhang et al. 2012). Interestingly, the ubiquitination status also plays a critical role in determination of cell fate. P53 poly-ubiquitination, which requires a polymeric ubiquitin chain, targets p53 for efficient proteasomal degradation, whereas mono-ubiquitination, which conjugates with a ubiquitin monomer, promotes the nuclear export or mitochondrial translocation of p53 (Li et al. 2003; Marchenko et al. 2007). Monoubiquitinated p53 favor cytoplasmic localization and functions as a ‘BH3-only’ (Willis and Adams 2005) to promote a first wave of apoptosis. A second wave of apoptosis is fulfilled by nuclear p53 as a transcription factor (Tian et al. 2012). MiR-605 and miR-34a, two p53 downstream transcriptional targets, can enhance the tumor suppressive function of p53 (Fig. 3e) (Zhou et al. 2014). Higher levels of DSBs will augment the effect of miR-605 and miR-34a to eradicate a cell in silico. Similarly, higher DNA damage favors homeodomain-interacting protein kinase 2 (HIPK2)-mediated Ser46 phosphorylation of p53 and results in concomitant decrease in MDM2 through multiple mechanisms leading to apoptosis (Fig. 3e) (Zhang et al. 2014). For severe DNA lesions, Tip-60 can acetylate p53 at lysine 120 (K120) through PUMA to significantly elevate p53 expression and trigger apoptosis (Fig. 3e) (Wang et al. 2019a). Equivalent modeling framework was also used in hypoxia induced cell fate decision through HIF-1 (Wang et al. 2019b). These mathematical models provide various ways to differentiate low and high levels of DNA damage in p53 signaling albeit with different cofactors. The major drawback in most p53 models for cell fate decision, however, is lack of direct experimental support although to some extent these experimental designs are infeasible. Moreover, p53 is massively post-translationally modified, which conferring complex specificity in p53 activities (Hafner et al. 2019; Batchelor and Loewer 2017). Most early models, however, only considered a minimal class of p53 modifications with defined roles in induction of important regulators of terminal cell fate (e.g. p21, PUMA). Cellular outcomes are more likely ascribed to coordinated expression of multiple genes. How p53 dynamics affects specific gene expression programs has not been resolved in these models.
It is noteworthy that p53 dynamics also influences cell fate in addition to the absolute level of p53 proteins. Due to the heterogeneity in p53 abundance in response to DNA damage, the mechanism connects temporal p53 patterns to specific gene expression remain open a challenge. By intricate manipulation in p53 dynamics, the p53 pulses were converted into a sustained plateau by Nutlin-3 treatment (Purvis et al. 2012). As a result, cell cycle progression cannot be recovered and commitment to senescence is usually observed if p53 undergoes sustained activation (Purvis et al. 2012). This prominent work has shed new light on how p53 dynamics per se affect terminal fate when cells are challenged by DNA damage.
An early model describes the diversity in p53 target gene expression was proposed by Batchelor and colleagues (Porter et al. 2016). The specificity in downstream target expression is primarily determined by mRNA half-life (Fig. 4a). When the half-life of a p53 target is lower than p53 protein, it can reflect the frequency information of p53 signals. On the contrary, mRNAs with slow decay rates can filter out the frequency aspects of p53 pulses and become steadily accumulated following each p53 pulse. Consequently, the p53 system behaves like a low-pass filter and p53 pulses limit the coordination in target gene expression (Porter et al. 2016). Furthermore, it seems that p53 targets will be induced following a ‘affinity model’, in which genes associated with cell cycle arrest are preferentially induced with higher p53 binding affinity (Weinberg et al. 2005; Murray-Zmijewski et al. 2008). Recent work by Lahav and colleagues have challenged this model (Hafner et al. 2017). Using chromatin immunoprecipitation sequencing (ChIP-seq), RNA sequencing (RNA-seq) measurements and mathematical modeling, they observed that p53 binding to target genomic foci is uniform albeit distinct profiles of p53 target gene expression. Clustering analysis has suggested that the dynamic induction patterns of p53 targets are not significantly different between cell cycle arrest and apoptotic genes. Surprisingly, the mRNA decay rates are responsible for the diversity in gene expressions (Hafner et al. 2017). Measurements of nascent mRNAs may provide additional information about dynamic decoding among p53 targets (Allen et al. 2014). In addition to mRNA stability, the protein stability also significantly contributes to the expression profiles of p53 targets. Batchelor and colleagues recently showed that distinct target gene expression patterns are produced by the relationship among p53 pulsing frequency, target mRNA and protein decay rates (Fig. 4b) (Hanson et al. 2019). By manipulating the stability of p53 targets, the expression profiles of p53 responses and cell fate in DDR can be changed. These studies collectively demonstrated the important role of timing control in p53 targets in DDR network.
Fig. 4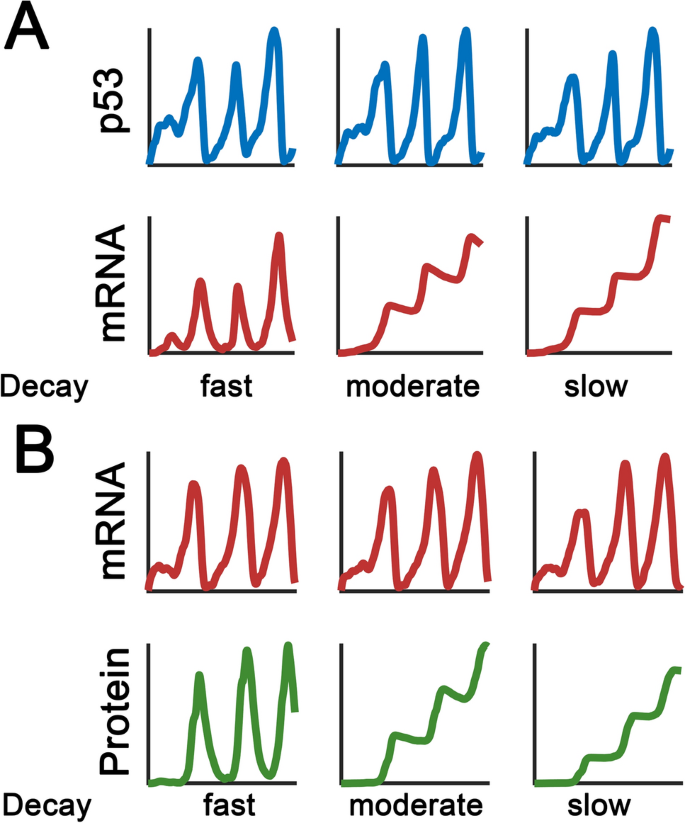
Decay rates affect the expression pattern of p53 targets. a Qualitatively similar p53 dynamics lead to different transcript expression patterns. The decay rates are varied as indicated. If a p53 target mRNA is slowly degraded, the mRNA becomes steadily accumulated after each p53 pulse. However, if the mRNA is fast-degrading, the p53 target mRNA can mirror the p53 dynamics with a certain delay. b The effect of protein stability on expression profiles. Similar mRNA profiles are generated. Fast-degrading proteins can follow the mRNA dynamics, whereas stable proteins undergo sustained elevation
Full size imageManipulation of p53 dynamics in cell fate decision
Significant variations in p53 dynamics have been observed using fluorescence reporter assays (Geva-Zatorsky et al. 2006). The connection between p53 dynamics and cellular effects have also been extensively explored in experiments and theoretical models (Batchelor and Loewer 2017; Hafner et al. 2019). A compelling idea argues that if p53 dynamics is manipulated even in the absence of DNA damage, similar cellular outcomes should be obtained. Recently, Batchelor and colleagues developed a method to alter p53 dynamics based on Nutlin-3, a chemical strategy to disrupt MDM2 and p53 binding (Harton et al. 2019; Vassilev et al. 2004). The p53 dynamics were reshaped into pulses with defined amplitude and duration (low, natural and high frequency p53 pulses). They next investigated how amplitude and temporal modulation affected two canonically regulated p53 targets, MDM2 and CDKN1A. Results suggested that MDM2 and CDKN1A promoters responded differentially. MDM2 promoter activation was most significant at natural p53 frequency (~ 5.5 h), whereas CDKN1A promoter was refractory to low frequency p53 pulses. Furthermore, the p53 pulses created a refractory duration for re-initiating a second pulse and sometimes behaves as a low-pass filter. Collectively, the p53 network can also leverage the diversity in target gene promoter activity to elicit different patterns of target gene expression.
Manipulation of p53 dynamics is also applied in a previous work by Lahav and colleagues using Nutlin-3 (Purvis et al. 2012). In their work, p53 became sustained activation with a plateau equivalent to p53 pulse peaks. Pulsatile or sustained p53 dynamics led to survival or permanent cell cycle arrest (senescence), respectively, albeit with similarly integrated p53 levels. Therefore, p53 dynamics per se can control cell fate. Lahav and colleagues suggested that feedforward regulation may be responsible for the bipartite responses. Notably, no mathematical models have been constructed to unravel the mechanism of such behaviors. These two prominent studies have provided remarkable clues to pharmacological manipulation of p53 dynamics especially in cancer treatments.
In addition, the oligomerization status of p53 may also contribute to cell fate decision. Fischer et al. used different oligomeric variants to generate monomeric, dimeric or tetrameric forms of p53 (Fischer et al. 2016). The dimeric p53 variants arrested cell growth, but failed to trigger apoptosis. In contrast, p53 tetramers induce rapid apoptosis and cell growth arrest, whereas the monomeric variant is functionally inactive (Fischer et al. 2016).
Conclusions
Despite 2 decades of theoretical studies in p53 signaling, our current knowledge about p53 functions is still largely uncertain. Most p53 models on cell fate decision depend heavily on the differential expression of critical effectors in p53 pathway with limited experimental supports. Whether the miscellaneous feedback loops or regulatory effects co-exist or behave in a cell type/stimulus-dependent manner remains to be defined. It is an interesting issue to investigate how pathway interactions can be translated into cell fate decision. A recent report showed that DNA damage elicits opposing pro-survival and pro-arrest signals via mitogen-activated protein kinase (MAPK) or p53, respectively (De et al. 2020). Sustained MAPK activation favors evasion from prolonged cell cycle arrest, whereas enhanced p53 activity halts cell cycle progression. More sophisticated p53 models are required to shed light on these issues. Furthermore, the spatial–temporal models of p53 dynamics are still limited (Gordon et al. 2009). In addition to mass action kinetics, Boolean or Markov network approach may also help to elucidate the dynamic properties of p53 signaling under conditions of insufficient quantitative measurements (Ge and Qian 2009; Liu and He 2016; Choi et al. 2012; Lin et al. 2014). The spatial constraints should be taken into account in physiologically cellular environment. The diverse post-translational controls and their coordinated roles for p53 are less investigated especially in mathematical models. High-throughput measurements together with detailed models will be helpful to unravel the encoding and decoding mechanisms in p53 signaling network.
References
Abou-Jaoude, W., Chaves, M., & Gouze, J. L. (2011). A theoretical exploration of birhythmicity in the p53-Mdm2 network. PLoS ONE, 6, e17075.
Allen, M. A., Andrysik, Z., Dengler, V. L., Mellert, H. S., Guarnieri, A., Freeman, J. A., et al. (2014). Global analysis of p53-regulated transcription identifies its direct targets and unexpected regulatory mechanisms. Elife, 3, e02200.
Bakkenist, C. J., & Kastan, M. B. (2003). DNA damage activates ATM through intermolecular autophosphorylation and dimer dissociation. Nature, 421, 499–506.
Banin, S., Moyal, L., Shieh, S., Taya, Y., Anderson, C. W., Chessa, L., et al. (1998). Enhanced phosphorylation of p53 by ATM in response to DNA damage. Science, 281, 1674–1677.
Batchelor, E., & Loewer, A. (2017). Recent progress and open challenges in modeling p53 dynamics in single cells. Current Opinion on System Biology, 3, 54–59.
Batchelor, E., Loewer, A., Mock, C., & Lahav, G. (2011). Stimulus-dependent dynamics of p53 in single cells. Molecular System Biology, 7, 488.
Batchelor, E., Mock, C. S., Bhan, I., Loewer, A., & Lahav, G. (2008). Recurrent initiation: A mechanism for triggering p53 pulses in response to DNA damage. Molecular Cell, 30, 277–289.
Bose, I., & Ghosh, B. (2007). The p53-MDM2 network: From oscillations to apoptosis. Journal of Biosciences, 32, 991–997.
Bottani, S., & Grammaticos, B. (2007). Analysis of a minimal model for p53 oscillations. Journal of Theoretical Biology, 249, 235–245.
Brooks, C. L., & Gu, W. (2006). p53 ubiquitination: Mdm2 and beyond. Molecular Cell, 21, 307–315.
Cai, X., & Yuan, Z. M. (2009). Stochastic modeling and simulation of the p53-MDM2/MDMX loop. Journal of Computational Biology, 16, 917–933.
Chen, X., Chen, J., Gan, S., Guan, H., Zhou, Y., Ouyang, Q., et al. (2013). DNA damage strength modulates a bimodal switch of p53 dynamics for cell-fate control. BMC Biology, 11, 73.
Chen, J., Yue, H., & Ouyang, Q. (2014). Correlation between oncogenic mutations and parameter sensitivity of the apoptosis pathway model. PLoS Computational Biology, 10, e1003451.
Cheng, Q., Chen, L., Li, Z., Lane, W. S., & Chen, J. (2009). ATM activates p53 by regulating MDM2 oligomerization and E3 processivity. EMBO Journal, 28, 3857–3867.
Cheng, Z., Ke, Y., Ding, X., Wang, F., Wang, H., Wang, W., et al. (2008). Functional characterization of TIP60 sumoylation in UV-irradiated DNA damage response. Oncogene, 27, 931–941.
Chipuk, J. E., Bouchier-Hayes, L., Kuwana, T., Newmeyer, D. D., & Green, D. R. (2005). PUMA couples the nuclear and cytoplasmic proapoptotic function of p53. Science, 309, 1732–1735.
Choi, M., Shi, J., Jung, S. H., Chen, X., & Cho, K. H. (2012). Attractor landscape analysis reveals feedback loops in the p53 network that control the cellular response to DNA damage. Scientific Signal, 5, ra83.
Chong, K. H., Samarasinghe, S., & Kulasiri, D. (2015). Mathematical modelling of p53 basal dynamics and DNA damage response. Mathematical Biosciences, 259, 27–42.
Ciliberto, A., Novak, B., & Tyson, J. J. (2005). Steady states and oscillations in the p53/Mdm2 network. Cell Cycle, 4, 488–493.
De, S., Campbell, C., Venkitaraman, A. R., & Esposito, A. (2020). Pulsatile MAPK signaling modulates p53 activity to control cell fate decisions at the g2 checkpoint for DNA damage. Cell Reports, 30(2083–2093), e2085.
Elias, J. (2017). Positive effect of Mdm2 on p53 expression explains excitability of p53 in response to DNA damage. Journal of Theoretical Biology, 418, 94–104.
Finzel, A., Grybowski, A., Strasen, J., Cristiano, E., & Loewer, A. (2016). Hyperactivation of ATM upon DNA-PKcs inhibition modulates p53 dynamics and cell fate in response to DNA damage. Molecular Biology of the Cell, 27, 2360–2367.
Fischer, N. W., Prodeus, A., Malkin, D., & Gariepy, J. (2016). p53 oligomerization status modulates cell fate decisions between growth, arrest and apoptosis. Cell Cycle, 15, 3210–3219.
Gajjar, M., Candeias, M. M., Malbert-Colas, L., Mazars, A., Fujita, J., Olivares-Illana, V., et al. (2012). The p53 mRNA-Mdm2 interaction controls Mdm2 nuclear trafficking and is required for p53 activation following DNA damage. Cancer Cell, 21, 25–35.
Gao, Y., Ferguson, D. O., Xie, W., Manis, J. P., Sekiguchi, J., Frank, K. M., et al. (2000). Interplay of p53 and DNA-repair protein XRCC4 in tumorigenesis, genomic stability and development. Nature, 404, 897–900.
Gao, C., Liu, H., & Yan, F. (2020). Dynamic behavior of p53 driven by delay and a microrna-34a-mediated feedback loop. International Journal of Molecular Science, 21, 1271.
Ge, H., & Qian, M. (2009). Boolean network approach to negative feedback loops of the p53 pathways: Synchronized dynamics and stochastic limit cycles. Journal of Computational Biology, 16, 119–132.
Geva-Zatorsky, N., Dekel, E., Batchelor, E., Lahav, G., & Alon, U. (2010). Fourier analysis and systems identification of the p53 feedback loop. Proceedings of National Academy of Science United States of America, 107, 13550–13555.
Geva-Zatorsky, N., Rosenfeld, N., Itzkovitz, S., Milo, R., Sigal, A., Dekel, E., et al. (2006). Oscillations and variability in the p53 system. Molecular System Biology, 2(2006), 0033.
Gordon, K. E., Van Leeuwen, I., Lain, S., & Chaplain, M. A. J. J. M. M. O. N. P. (2009). Spatio-temporal modelling of the p53–mdm2 oscillatory system. Journal of Theoretical Biology, 4, 97–116.
Green, D. R., & Kroemer, G. (2009). Cytoplasmic functions of the tumour suppressor p53. Nature, 458, 1127–1130.
Hafner, A., Bulyk, M. L., Jambhekar, A., & Lahav, G. (2019). The multiple mechanisms that regulate p53 activity and cell fate. Nature Reviews Molecular Cell Biology, 20, 199–210.
Hafner, A., Stewart-Ornstein, J., Purvis, J. E., Forrester, W. C., Bulyk, M. L., & Lahav, G. (2017). p53 pulses lead to distinct patterns of gene expression albeit similar DNA-binding dynamics. Nature Structural and Molecular Biology, 24, 840–847.
Hanson, R. L., Porter, J. R., & Batchelor, E. (2019). Protein stability of p53 targets determines their temporal expression dynamics in response to p53 pulsing. Journal of Cell Biology, 218, 1282–1297.
Harris, S. L., & Levine, A. J. (2005). The p53 pathway: Positive and negative feedback loops. Oncogene, 24, 2899–2908.
Harton, M. D., Koh, W. S., Bunker, A. D., Singh, A., & Batchelor, E. (2019). p53 pulse modulation differentially regulates target gene promoters to regulate cell fate decisions. Molecular System Biology, 15, e8685.
Hemann, M. T., & Lowe, S. W. (2006). The p53-Bcl-2 connection. Cell Death and Differentiation, 13, 1256–1259.
Hengartner, M. O. (2000). The biochemistry of apoptosis. Nature, 407, 770–776.
Hu, W., Feng, Z., Ma, L., Wagner, J., Rice, J. J., Stolovitzky, G., et al. (2007). A single nucleotide polymorphism in the MDM2 gene disrupts the oscillation of p53 and MDM2 levels in cells. Cancer Research, 67, 2757–2765.
Hunziker, A., Jensen, M. H., & Krishna, S. (2010). Stress-specific response of the p53-Mdm2 feedback loop. BMC Systems Biology, 4, 94.
Ichijima, Y., Sakasai, R., Okita, N., Asahina, K., Mizutani, S., & Teraoka, H. (2005). Phosphorylation of histone H2AX at M phase in human cells without DNA damage response. Biochemical and Biophysical Research Communications, 336, 807–812.
Iwamoto, K., Hamada, H., Eguchi, Y., & Okamoto, M. (2011). Mathematical modeling of cell cycle regulation in response to DNA damage: Exploring mechanisms of cell-fate determination. Biosystems, 103, 384–391.
Jolma, I. W., Ni, X. Y., Rensing, L., & Ruoff, P. J. B. J. (2010). Harmonic oscillations in homeostatic controllers: Dynamics of the p53 regulatory system. Biophysical Journal, 98, 743–752.
Jones, S. N., Roe, A. E., Donehower, L. A., & Bradley, A. (1995). Rescue of embryonic lethality in Mdm2-deficient mice by absence of p53. Nature, 378, 206–208.
Karanam, K., Kafri, R., Loewer, A., & Lahav, G. (2012). Quantitative live cell imaging reveals a gradual shift between DNA repair mechanisms and a maximal use of HR in mid S phase. Molecular Cell, 47, 320–329.
Kim, J. K., & Jackson, T. L. J. P. O. (2013). Mechanisms that enhance sustainability of p53 pulses. PLoS One, 8, e65242.
Krenning, L., van den Berg, J., & Medema, R. H. (2019). Life or death after a break: What determines the choice? Molecular Cell, 76, 346–358.
Kultz, D. (2005). Molecular and evolutionary basis of the cellular stress response. Annual Review of Physiology, 67, 225–257.
Lahav, G., Rosenfeld, N., Sigal, A., Geva-Zatorsky, N., Levine, A. J., Elowitz, M. B., et al. (2004). Dynamics of the p53-Mdm2 feedback loop in individual cells. Nature Genetics, 36, 147–150.
Lane, D. P. (1992). Cancer. p53, guardian of the genome. Nature, 358, 15–16.
Lecona, E., & Fernandez-Capetillo, O. (2018). Targeting ATR in cancer. Nature Reviews Cancer, 18, 586–595.
Lev Bar-Or, R., Maya, R., Segel, L. A., Alon, U., Levine, A. J., & Oren, M. (2000). Generation of oscillations by the p53-Mdm2 feedback loop: A theoretical and experimental study. Proceedings of National Academy of Science United States of America, 97, 11250–11255.
Li, M., Brooks, C. L., Wu-Baer, F., Chen, D., Baer, R., & Gu, W. (2003). Mono-versus polyubiquitination: Differential control of p53 fate by Mdm2. Science, 302, 1972–1975.
Lin, G., Ao, B., Chen, J., Wang, W., & Di, Z. (2014). Modeling and controlling the two-phase dynamics of the p53 network: A Boolean network approach. New Journal of Physics, 16, 125010.
Liu, B., Bhatt, D., Oltvai, Z. N., Greenberger, J. S., & Bahar, I. (2014). Significance of p53 dynamics in regulating apoptosis in response to ionizing radiation, and polypharmacological strategies. Science Reports, 4, 6245.
Liu, Q., & He, Z. (2016). A novel boolean network for analyzing the p53 gene regulatory network. Current Bioinformatics, 11, 13–21.
Loewer, A., Batchelor, E., Gaglia, G., & Lahav, G. (2010). Basal dynamics of p53 reveal transcriptionally attenuated pulses in cycling cells. Cell, 142, 89–100.
Loewer, A., Karanam, K., Mock, C., & Lahav, G. (2013). The p53 response in single cells is linearly correlated to the number of DNA breaks without a distinct threshold. BMC Biology, 11, 114.
Lowndes, N. F. (2001). DNA-damage signaling and apoptosis. Genome Biology, 2, REPORTS4028.
Ma, A., & Dai, X. J. C. C. (2018). The relationship between DNA single-stranded damage response and double-stranded damage response. Cell Cycle, 17, 73–79.
Ma, A., & Dai, X. (2019). Exploring the influence of parameters on the p53 response when single-stranded breaks and double-stranded breaks coexist. Interdisciplinary Sciences: Computational Life Sciences, 11, 679–690.
Ma, L., Wagner, J., Rice, J. J., Hu, W., Levine, A. J., & Stolovitzky, G. A. (2005). A plausible model for the digital response of p53 to DNA damage. Proceedings of National Academy of Science United States of America, 102, 14266–14271.
Malbert-Colas, L., Ponnuswamy, A., Olivares-Illana, V., Tournillon, A. S., Naski, N., & Fahraeus, R. (2014). HDMX folds the nascent p53 mRNA following activation by the ATM kinase. Molecular Cell, 54, 500–511.
Marchenko, N. D., Wolff, S., Erster, S., Becker, K., & Moll, U. M. (2007). Monoubiquitylation promotes mitochondrial p53 translocation. EMBO Journal, 26, 923–934.
Monk, N. A. (2003). Oscillatory expression of Hes1, p53, and NF-kappaB driven by transcriptional time delays. Current Biology, 13, 1409–1413.
Monke, G., Cristiano, E., Finzel, A., Friedrich, D., Herzel, H., Falcke, M., et al. (2017). Excitability in the p53 network mediates robust signaling with tunable activation thresholds in single cells. Science Reports, 7, 46571.
Moore, R., Ooi, H. K., Kang, T., Bleris, L., & Ma, L. (2015). MiR-192-mediated positive feedback loop controls the robustness of stress-induced p53 oscillations in breast cancer cells. PLoS Computational Biology, 11, e1004653.
Mouri, K., Nacher, J. C., & Akutsu, T. (2009). A mathematical model for the detection mechanism of DNA double-strand breaks depending on autophosphorylation of ATM. PLoS One, 4, e5131.
Muller, P. A., & Vousden, K. H. (2013). p53 mutations in cancer. Nature Cell Biology, 15, 2–8.
Murray-Zmijewski, F., Slee, E. A., & Lu, X. (2008). A complex barcode underlies the heterogeneous response of p53 to stress. Nature Reviews Molecular Cell Biology, 9, 702–712.
Nakano, K., & Vousden, K. H. (2001). PUMA, a novel proapoptotic gene, is induced by p53. Molecular Cell, 7, 683–694.
Park, J. H., Yang, S. W., Park, J. M., Ka, S. H., Kim, J. H., Kong, Y. Y., et al. (2016). Positive feedback regulation of p53 transactivity by DNA damage-induced ISG15 modification. Nature Communications, 7, 12513.
Porter, J. R., Fisher, B. E., & Batchelor, E. (2016). p53 pulses diversify target gene expression dynamics in an mRNA half-life-dependent manner and delineate co-regulated target gene subnetworks. Cell System, 2, 272–282.
Proctor, C. J., & Gray, D. A. (2008). Explaining oscillations and variability in the p53-Mdm2 system. BMC Systems Biology, 2, 75.
Pu, T., Zhang, X. P., Liu, F., & Wang, W. (2010). Coordination of the nuclear and cytoplasmic activities of p53 in response to DNA damage. Biophysical Journal, 99, 1696–1705.
Purvis, J. E., Karhohs, K. W., Mock, C., Batchelor, E., Loewer, A., & Lahav, G. (2012). p53 dynamics control cell fate. Science, 336, 1440–1444.
Puszynski, K., Hat, B., & Lipniacki, T. (2008). Oscillations and bistability in the stochastic model of p53 regulation. Journal of Theoretical Biology, 254, 452–465.
Ramalingam, S., Honkanen, P., Young, L., Shimura, T., Austin, J., Steeg, P. S., et al. (2007). Quantitative assessment of the p53-Mdm2 feedback loop using protein lysate microarrays. Cancer Research, 67, 6247–6252.
Saldivar, J. C., Cortez, D., & Cimprich, K. A. (2017). The essential kinase ATR: Ensuring faithful duplication of a challenging genome. Nature Reviews Molecular Cell Biology, 18, 622–636.
Serrano, M. A., Li, Z., Dangeti, M., Musich, P. R., Patrick, S., Roginskaya, M., et al. (2013). DNA-PK, ATM and ATR collaboratively regulate p53-RPA interaction to facilitate homologous recombination DNA repair. Oncogene, 32, 2452–2462.
Shikazono, N., Noguchi, M., Fujii, K., Urushibara, A., & Yokoya, A. (2009). The yield, processing, and biological consequences of clustered DNA damage induced by ionizing radiation. Journal of Radiated Research, 50, 27–36.
Shimada, M., & Nakanishi, M. (2013). Response to DNA damage: Why do we need to focus on protein phosphatases? Frontiers Oncology, 3, 8.
So, A., Le Guen, T., Lopez, B. S., & Guirouilh-Barbat, J. (2017). Genomic rearrangements induced by unscheduled DNA double strand breaks in somatic mammalian cells. FEBS Journal, 284, 2324–2344.
Stewart-Ornstein, J., Cheng, H. W. J., & Lahav, G. (2017). Conservation and divergence of p53 oscillation dynamics across species. Cell System, 5(410–417), e414.
Stewart-Ornstein, J., & Lahav, G. (2017). p53 dynamics in response to DNA damage vary across cell lines and are shaped by efficiency of DNA repair and activity of the kinase ATM. Science Signaling, 10, eaah6671.
Su, T. T. (2006). Cellular responses to DNA damage: One signal, multiple choices. Annual Review of Genetics, 40, 187–208.
Sun, T., Chen, C., Wu, Y., Zhang, S., Cui, J., & Shen, P. (2009). Modeling the role of p53 pulses in DNA damage- induced cell death decision. BMC Bioinformatics, 10, 190.
Sun, T., & Cui, J. (2014). A plausible model for bimodal p53 switch in DNA damage response. FEBS Letters, 588, 815–821.
Sun, T., & Cui, J. (2015). Dynamics of P53 in response to DNA damage: Mathematical modeling and perspective. Progress in Biophysics and Molecular Biology, 119, 175–182.
Sun, T., Li, X., & Shen, P. (2017). Modeling amplified p53 responses under DNA-PK inhibition in DNA damage response. Oncotarget, 8, 17105–17114.
Sun, T., & Mu, D. (2020). Multi-scale modeling identifies the role of p53-Gys2 negative feedback in cellular homeostasis. Mathematical Biosciences and Engineering, 17, 3260–3273.
Sun, T., Mu, D., & Cui, J. (2018). Mathematical model identifies effective P53 accumulation with target gene binding affinity in DNA damage response for cell fate decision. Cell Cycle, 17, 2716–2730.
Sun, T., Yang, W., Liu, J., & Shen, P. (2011). Modeling the basal dynamics of P53 system. PLoS One, 6, e27882.
Sun, T., Yuan, R., Xu, W., Zhu, F., & Shen, P. (2010). Exploring a minimal two-component p53 model. Physical Biology, 7, 036008.
Tang, Q., Su, Z., Gu, W., & Rustgi, A. K. (2020). Mutant p53 on the path to metastasis. Trends Cancer, 6, 62–73.
Tian, X. J., Liu, F., Zhang, X. P., Li, J., & Wang, W. (2012). A two-step mechanism for cell fate decision by coordination of nuclear and mitochondrial p53 activities. PLoS ONE, 7, e38164.
Tiana, G., Jensen, M. H., & Sneppen, K. J. E. P. J. B. (2002). Time delay as a key to apoptosis induction in the p53 network. The European Physical Journal B-Condensed Matter and Complex, 29, 135–140.
Tibbetts, R. S., Brumbaugh, K. M., Williams, J. M., Sarkaria, J. N., Cliby, W. A., Shieh, S. Y., et al. (1999). A role for ATR in the DNA damage-induced phosphorylation of p53. Genes and Development, 13, 152–157.
Toettcher, J. E., Loewer, A., Ostheimer, G. J., Yaffe, M. B., Tidor, B., & Lahav, G. (2009). Distinct mechanisms act in concert to mediate cell cycle arrest. Proceedings of National Academy of Science United States of America, 106, 785–790.
Tyson, J. (2006). Another turn for p53. Molecular Systems Biology, 2(2006), 0032.
Tyson, J. J., Chen, K. C., & Novak, B. (2003). Sniffers, buzzers, toggles and blinkers: Dynamics of regulatory and signaling pathways in the cell. Current Opinion in Cell Biology, 15, 221–231.
Unsal-Kacmaz, K., Makhov, A. M., Griffith, J. D., & Sancar, A. (2002). Preferential binding of ATR protein to UV-damaged DNA. Proceedings of National Academy of Science United States of America, 99, 6673–6678.
Vassilev, L. T., Vu, B. T., Graves, B., Carvajal, D., Podlaski, F., Filipovic, Z., et al. (2004). In vivo activation of the p53 pathway by small-molecule antagonists of MDM2. Science, 303, 844–848.
Vilenchik, M. M., & Knudson, A. G. (2003). Endogenous DNA double-strand breaks: Production, fidelity of repair, and induction of cancer. Proceedings of the National academy of Sciences of the United States of America, 100, 12871–12876.
Wagner, J., Ma, L., Rice, J. J., Hu, W., Levine, A. J., & Stolovitzky, G. A. (2005). p53-Mdm2 loop controlled by a balance of its feedback strength and effective dampening using ATM and delayed feedback. Systematic Biology (Stevenage), 152, 109–118.
Wang, P., Bao, H., Zhang, X. P., Liu, F., & Wang, W. (2019a). Regulation of Tip60-dependent p53 acetylation in cell fate decision. FEBS Letters, 593, 13–22.
Wang, P., Guan, D., Zhang, X. P., Liu, F., & Wang, W. (2019b). Modeling the regulation of p53 activation by HIF-1 upon hypoxia. FEBS Letters, 593, 2596–2611.
Wang, L. S., Li, N. X., Chen, J. J., Zhang, X. P., Liu, F., & Wang, W. (2018). Modulation of dynamic modes by interplay between positive and negative feedback loops in gene regulatory networks. Physical Review E, 97, 042412.
Wee, K. B., Surana, U., & Aguda, B. D. (2009). Oscillations of the p53-Akt network: Implications on cell survival and death. PLoS One, 4, e4407.
Weinberg, R. L., Veprintsev, D. B., Bycroft, M., & Fersht, A. R. (2005). Comparative binding of p53 to its promoter and DNA recognition elements. Journal of Molecular Biology, 348, 589–596.
Willis, S. N., & Adams, J. M. (2005). Life in the balance: How BH3-only proteins induce apoptosis. Current Opinion in Cell Biology, 17, 617–625.
Wu, X., Bayle, J. H., Olson, D., & Levine, A. J. (1993). The p53-mdm-2 autoregulatory feedback loop. Genes and Development, 7, 1126–1132.
Wu, W., Sun, W., & Sun, T. (2016). Modeling the heterogeneity of p53 dynamics in DNA damage response. Journal of Bioinformatics and Computational Biology, 14, 1650001.
Wu, M., Ye, H., Tang, Z., Shao, C., Lu, G., Chen, B., et al. (2017). p53 dynamics orchestrates with binding affinity to target genes for cell fate decision. Cell Death and Disease, 8, e3130.
Xu, L., Chen, Y., Song, Q., Xu, D., Wang, Y., & Ma, D. (2009). PDCD5 interacts with Tip60 and functions as a cooperator in acetyltransferase activity and DNA damage-induced apoptosis. Neoplasia, 11, 345–354.
Yang, R., Huang, B., Zhu, Y., Li, Y., Liu, F., & Shi, J. (2018). Cell type-dependent bimodal p53 activation engenders a dynamic mechanism of chemoresistance. Scientific Advances, 4, eaat5077.
Zhang, T., Brazhnik, P., & Tyson, J. J. (2007). Exploring mechanisms of the DNA-damage response: p53 pulses and their possible relevance to apoptosis. Cell Cycle, 6, 85–94.
Zhang, T., Brazhnik, P., & Tyson, J. J. (2009a). Computational analysis of dynamical responses to the intrinsic pathway of programmed cell death. Biophysical Journal, 97, 415–434.
Zhang, X., Liu, F., Cheng, Z., & Wang, W. (2009b). Cell fate decision mediated by p53 pulses. Proceedings of National Academy of Science United States of America, 106, 12245–12250.
Zhang, X., Liu, F., & Wang, W. (2010). Coordination between cell cycle progression and cell fate decision by the p53 and E2F1 pathways in response to DNA damage. Journal of Biological Chemistry, 285, 31571–31580.
Zhang, X., Liu, F., & Wang, W. (2011). Two-phase dynamics of p53 in the DNA damage response. Proceedings of National Academy of Science United States of America, 108, 8990–8995.
Zhang, X., Liu, F., & Wang, W. (2012). Regulation of the DNA damage response by p53 cofactors. Biophysical Journal, 102, 2251–2260.
Zhang, X., Liu, F., & Wang, W. (2014). Interplay between Mdm2 and HIPK2 in the DNA damage response. Journal of The Royal Society Interface, 11, 20140319.
Zhou, Y., Lee, J. H., Jiang, W., Crowe, J. L., Zha, S., & Paull, T. T. (2017). Regulation of the DNA damage response by DNA-PKcs inhibitory phosphorylation of ATM. Molecular Cell, 65, 91–104.
Zhou, C. H., Zhang, X. P., Liu, F., & Wang, W. (2014). Involvement of miR-605 and miR-34a in the DNA damage response promotes apoptosis induction. Biophysical Journal, 106, 1792–1800.
Zhuge, C., Chang, Y., Li, Y., Chen, Y., & Lei, J. (2011). PDCD5-regulated cell fate decision after ultraviolet-irradiation-induced DNA damage. Biophysical Journal, 101, 2582–2591.
Zhuge, C., Sun, X., Chen, Y., & Lei, J. (2016). PDCD5 functions as a regulator of p53 dynamics in the DNA damage response. Journal of Theoretical Biology, 388, 1–10.
Funding
This work is supported by the National Key Research and Development Program of China (2017YFA0506000, 2017YFA0205400), National Natural Science Foundation of China (31971185).
Author information
Affiliations
State Key Laboratory of Pharmaceutical Biotechnology and The Comprehensive Cancer Center, Nanjing Drum Tower Hospital, MOE Key Laboratory of Model Animal for Disease Study, School of Life Sciences, Nanjing University, Nanjing, 210046, China
Nanfei Yang & Pingping Shen
School of Life Sciences, Anqing Normal University, North Jixian Road, No. 1318, Anqing, 246133, Anhui, China
Tingzhe Sun
Corresponding authors
Correspondence to Tingzhe Sun or Pingping Shen.
Ethics declarations
Conflict of interest
The authors declare that there is no conflict of interest.
Rights and permissions
About this article
Cite this article
Yang, N., Sun, T. & Shen, P. Deciphering p53 dynamics and cell fate in DNA damage response using mathematical modeling. GENOME INSTAB. DIS. 1, 265–277 (2020). https://doi.org/10.1007/s42764-020-00019-6
Received 31 May 2020
Revised 01 July 2020
Accepted 11 July 2020
Published 18 July 2020
Issue Date September 2020
Share this article
Anyone you share the following link with will be able to read this content:
Get shareable linkKeywords
p53
Mathematical model
Feedback loop
Cell fate decision
用户登录
还没有账号?
立即注册