Cell plasticity and genomic instability in cancer evolution
Review Article
Yan Xu, Hui Li, Fan Yang, Dingpeng Yang & Bin-Bing S. Zhou
Genome Instability & Disease (2020)
Abstract
Cancer cells evolve throughout disease progression and tumor relapse. Such evolution is a dynamic process resulting in genotypic and phenotypic cellular changes, conferring a high level of cell plasticity. Data derived from next-generation sequencing strategies have implicated that cancer cell plasticity could be driven by genetic changes induced by genomic instability during cancer evolution. Understanding the mechanisms of how genomic instability promotes mutagenesis and cancer cell plasticity could thus be critical avenue for cancer prevention and intervention. In this review, we discuss the relationships between cancer cell plasticity, genomic instability and mutagenesis during cancer evolution. We offer our insight and opinion on therapeutic strategies in this rapidly progressing research field.
Introduction
Cancer derives from aberrantly proliferating cells that can avoid cell death and persist despite an accumulation of genetic mutations. After initial malignant transformation, cancer cells continue to evolve during disease progression and tumor relapse (Alexandrov et al. 2020; Lipinski et al. 2016).
As a committed champion of evolutionism, Dr. Peter Nowell proposed the cancer clonal evolution theory, whereby cancer clones with a survival advantage can prevail under selection pressure (Nowell 1976). Similar to the classical species evolution model, cancer evolution is a dynamic process: various genotypic and phenotypic cellular changes can occur to confer a high degree of cell plasticity (Boumahdi and de Sauvage 2020; Yuan et al. 2019). As a main inducer of cancer heterogeneity and drug resistance, cell plasticity is driven by diverse causes, such as exogenous selection pressures (including environmental and treatment stress) and endogenous adaptability (including genetic and epigenetic instability) (Boumahdi and de Sauvage 2020; Yuan et al. 2019). Both oncogenes and tumor suppressors can affect cellular plasticity and adaptability. Data derived from next-generation sequencing approaches have implicated that cancer cell plasticity could be driven by numerous genetic changes during cancer evolution (Alexandrov et al. 2020; Ferrando and Lopez-Otin 2017) and revealed significant intra-tumoral heterogeneity in cancer cells. What’s more, genomic instability seems to not only change cellular phenotypes, but also alter cancer cell adaptability and plasticity in response to environmental changes and treatment pressures. In this review, we highlight the emerging connection between cancer cell plasticity, genomic instability and mutagenesis during cancer evolution, and offer our insight and opinion on direction and therapeutic strategies in this rapidly progressing research field.
Cell plasticity and genomic instability
As one of the major causes of tumor cell plasticity, genomic instability is the main driver of drug resistance and tumor relapse (Hanahan and Weinberg 2011). For instance, Mad2 overexpression induces chromosomal instability (e.g. aneuploidy) (Cahill et al. 1998), one type of genomic stability, in KRAS-driven lung cancer. Although removing KRAS results in remission, Mad2-induced genomic instability activates alternative signaling pathways, including ERK, AKT and STAT3, which release the dependency of cancer cells on KRAS and lead to a relapse (Sotillo et al. 2010). Mutation rates (a parameter of genomic instability) in colon cancer cells are increased by tumor-targeted therapies, which eventually lead to drug-resistant mutations (Misale et al. 2012; Russo et al. 2019; Diaz et al. 2012). Furthermore, synthesis of ErbB3 ligands and thus amplification of the ErbB3-related oncogenic signaling loop can elevate cell plasticity and result in target drug resistance (Zhou et al. 2006). These findings support that genomic instability and related cell plasticity drives tumor relapse and cancer evolution.
Selection pressure in cancer evolution could come not only from environmental changes that affect growth, but also drug-induced stresses. Genomic instability can affect plasticity and the adaptability of tumor cell clones and subclones during their evolution. Based on these features, the cancer evolution patterns during therapeutic treatment can be summarized into three models: (1) a therapy-induced mutation model, (2) a therapy-selection model, and (3) a de novo resistance model (Ferrando and Lopez-Otin 2017; Li et al. 2020). Therapeutic drugs, such as cisplatin (Boot et al. 2018), etoposide (Felix et al. 1995) and thiopurines (Li et al. 2020), could promote genomic instability and mutagenesis; here, the therapy-induced mutation model describes the scenario that no fully resistant subclone is present at diagnosis but rather therapy-induced drug-resistant mutations drive relapse. The therapy-selection model, however, describes that a minor drug-tolerant or resistant subclone survives therapy and acquires an additional mutation(s) and phenotype switches that lead to relapse (Kong et al. 2017; Okazaki et al. 2018).
Of note, epigenetic mechanisms could also confer the necessary cell plasticity for therapy selection, although this is not a focus of this review. In short, the promoter CpG island hypermethylation-associated silencing of the putative DNA/RNA helicase Schlafen-11 (SLFN11) is associated with an increased resistance to platinum compounds in ovarian and non-small cell lung cancers (Nogales et al. 2016). Unmethylated O6-methylguanine–DNA methyltransferase (MGMT) is associated with increased resistance to temozolomide (TMZ) in glioblastoma multiforme cancers (Kirstein et al. 2020).
In the de novo resistance model, most cells are therapy-resistant from the start, and remission is never achieved (Fig. 1). Thus, the therapy-induced mutation and therapy-selection models are the most relevant to genomic instability-driven drug resistance and cell plasticity in cancer evolution.
Fig. 1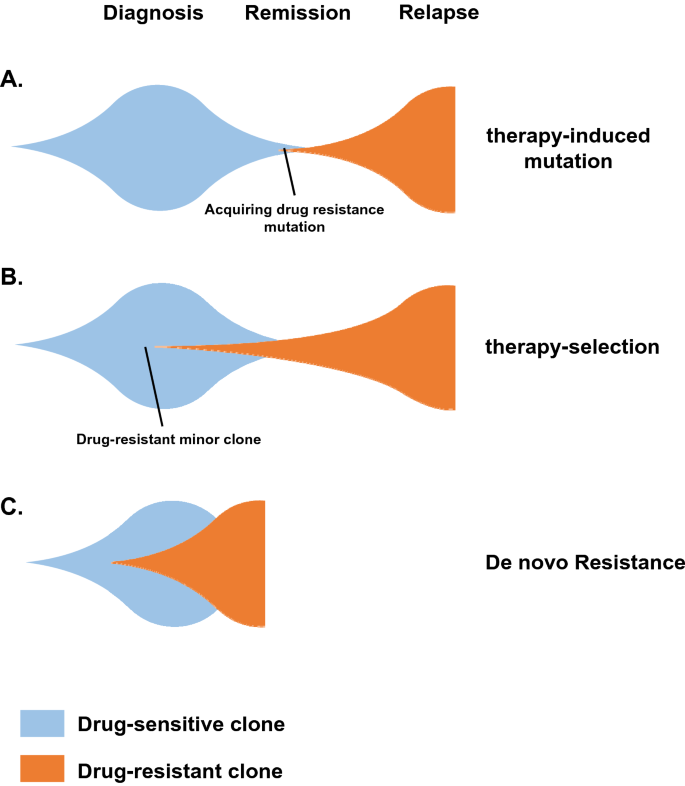
Models of cancer evolution during therapeutic treatment. a The therapy-induced mutation model describes the scenario that no fully resistant subclone is present at diagnosis and that rather, therapy-induced drug resistant mutations drive cancer relapse. b The therapy-selection model describes that a minority drug-tolerant subclone survives therapy and acquires additional mutation(s) that ultimately lead to cancer relapse. c In the de novo resistance model, most cells are initially therapy-resistant and remission is never achieved
Full size imageRegulation of genomic instability in cancer
As a hallmark of cancer, genomic instability can promote cell plasticity and improve cancer cell fitness during cancer evolution (Hanahan and Weinberg 2011). On the one hand, genomic instability can be induced by mutations and copy number variations of cancer genes (such as oncogenes and tumor suppressors); on the other hand, environmental stresses, especially therapeutic drug-induced stress, can also cause genomic instability and induce mutagenesis (Fig. 2). Organisms respond to endogenous and exogenous chromosomal insults by activating a complex DNA damage response (DDR) signaling pathway including sensors, transducers and effectors (Zhou and Elledge 2000), of which many are putative tumor suppressors. DNA damage sensors include MRN (MRE11-RAD50-NBS1) (Bian et al. 2019) and ATR-ARTIP complexes (Zou and Elledge 2003), and in some cases, mismatch repair (MMR) complexes. Transducers include the proximal kinases ATM and ATR, and the downstream kinases CHK1 and CHK2. Next are the DDR kinase substrates involved in DNA repair, transcription regulation, cell cycle control or apoptosis such as BRCA1, BRCA2, CDC25C and p53. Deficiencies in the DDR and DNA repair genes could cause different types of genomic instability that promotes mutation acquisition and malignant transformation. For instance, non-homologous end-joining (NHEJ) and alternative end-joining (alt-EJ) predominantly contribute to deletions and translocations in esophageal squamous cell carcinoma (ESCC) (Cheng et al. 2016). ATM and LIG4 prevent chromosome translocation from c-myc to the S region, and ATM and LIG4 deficiency increases microhomology-mediated double strand break (DSB) end-joining and in this way can cause genomic instability (Panchakshari et al. 2018). Mutations in DDR genes are associated with a high tumor mutational burden (Mouw et al. 2017; Rizvi et al. 2015; Chae et al. 2019), an indicator of genomic instability. An MMR deficiency could induce hereditary non-polyposis colon cancer (HNPCC) (Wheeler et al. 2000; Goodfellow et al. 2003). In addition, mutations in BRCA1 and BRCA2 of homologous recombination (HR) repair pathway have been identified in multiple cancers including hereditary thymic carcinoma (Morganella et al. 2016). Furthermore, whole genome sequencing of breast cancer samples showed the existence of an MMR or HR deficiency in most of them (Nik-Zainal et al. 2016). Similarly, a study of pancreatic cancer discovered that patients with defective MMR or HR suffered higher malignant levels and a worse prognosis than those with functional MMR and HR (Hu et al. 2018; Li et al. 2006).
Fig. 2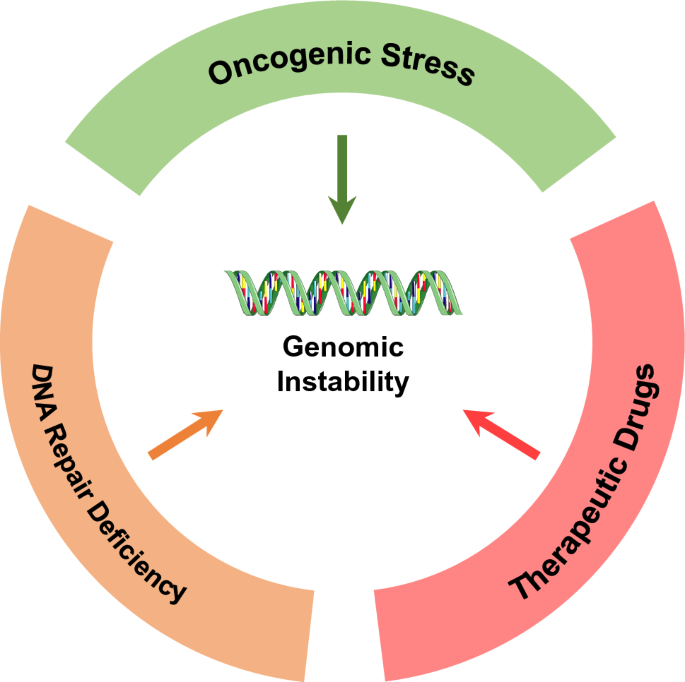
Drivers of genomic instability. Genomic instability can be induced by mutations and copy number variations of cancer genes, including oncogenes, tumor suppressors, and DNA repair genes. Environmental stresses, especially therapeutic drugs-induced stress, can also cause genomic instability
Full size imageOncogenes not only promote tumor cell proliferation: they also generate oncogenic stress leading to increased genomic instability (Halazonetis et al. 2008). Oncogenes can promote new firing at replication origins and exhaust the intracellular dNTP pool, which increases replication fork stalling and collapse leading to DNA DSBs (Venkitaraman 2011; Macheret and Halazonetis 2018). Oncogene-induced replication stress can also expose the single strand DNA that would usually be cleaved by endonucleases or be modified by the deaminases APOBEC3 (Alexandrov et al. 2013; Burns et al. 2013) or AID (Matsumoto et al. 2007). Moreover, aberrant replication can induce conflict between replication and transcription, which causes DSBs in DNA (Garcia-Muse and Aguilera 2016). Nevertheless, oncogene-induced genomic damage sites are not random: most of them occur at chromosomal fragile sites (Campbell et al. 2017).
Anti-cancer chemotherapeutic drugs include DNA alkylating agents, topoisomerase inhibitors, nucleoside analogues and microtubule inhibitors. Many of them can promote DNA DSBs, mutagenesis and the emergence of drug-resistant mutations (Kucab et al. 2019; Li et al. 2020). Recently, it was reported that targeted drugs can also cause replication stress and genomic instability (Russo et al. 2019; Juvekar et al. 2012; Ibrahim et al. 2012), similar to the antibiotic-induced stress response in bacteria (McKenzie and Rosenberg 2001). For example, the EGFR inhibitors gefitinib or erlotinib can cause an increase in copy number of EGFR localized on chromosome 7 and drug resistance in lung cancer cells (Bean et al. 2007). Therefore, both chemotherapeutic and targeted drugs have the potential to confer treatment-induced mutagenesis and selection pressure during cancer evolution.
Increased understanding of genomic instability by new methods
Understanding the critical role of genomic instability in cancer evolution relies on rapid and sensitive detection methods. As such, new technologies have been developed to detect DNA damage and genomic instability from the single cell level to a high-throughput format. Genomic instability can cause DNA damage and activate DDR signaling pathways (Zhou and Elledge 2000; Jeggo et al. 2016); therefore, markers of DDR activation (e.g. γ-H2AX) are typically used as sensitive biomarkers. Researchers have also developed methods to quantify the levels of DNA damage (e.g. by comet assay) and to identify the relative positions of damaged sites, for instance by Breaks Labeling in Situ and Sequencing (BLISS) (Crosetto et al. 2013) and END sequencing (END-seq) (Canela et al. 2016).
With the development of high-throughput and next-generation sequencing technologies, big data strategies have become a popular tool to systematically study genomic instability. Remarkably, Alexandrov et al. pioneered a mutational processes study, in which they found mutations in specific DNA sequences can be categorized as different mutational signatures (Alexandrov et al. 2013). Several mutational signatures have since been considered to be related with tissue specificity, DNA repair deficiency, age, carcinogen or chemotherapy (Yokoyama et al. 2019; Kucab et al. 2019; Boot et al. 2018; Martincorena et al. 2015), while others have been shown to underlie causal relationships (such as a drinking habit and alcohol dehydrogenase in esophagus cancer or UV in skin cancer) (Sawada et al. 2016). Another signature found in lung cancer cells reflected nicotine-induced mutagenesis (Alexandrov et al. 2016). Furthermore, chemotherapy-induced novel mutational signatures might generate drug-resistant mutations and drive clonal evolution, leading to acute lymphoblastic leukemia (ALL) relapse (Li et al. 2020). Although the molecular mechanisms of these signatures remain largely unknown, some mutagens or environmental factors have been associated with tumorigenesis and disease progression, providing new avenues for cancer prevention and intervention. There is no doubt that combining mutation signature discovery with functional analysis will provide not only a new approach to study the driving mechanisms underlying cancer evolution but also novel therapeutic strategies with better efficacy (Fig. 3).
Fig. 3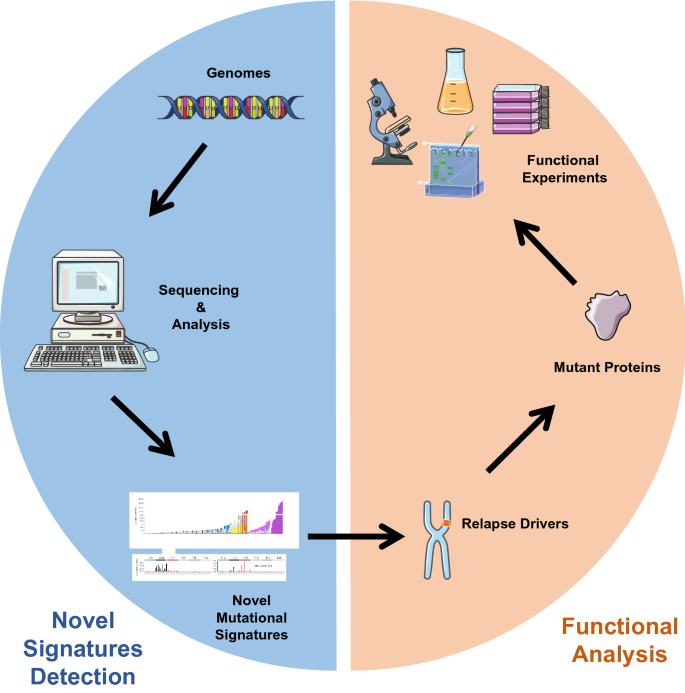
Combining novel mutation signature detection with functional analyses to determine the drivers of relapse. Novel mutation signatures detected using sequencing technologies could help researchers to reveal the relevance between known relapse drivers and certain mutagens or environmental factors. By this approach, it is also possible to identify potential unknown resistant mutations according to the mutation signatures. Functional analyses that follow could help experimentally link the mutagens and mutations, as well as discover the role of novel resistant mutations in cancer relapse and evolution
Full size imageTherapeutic strategies targeting genomic instability
Many of the current standard cancer therapies are DNA damaging chemotherapeutics, which can also damage normal tissues. Targeting the genomic instability features of cancer cells may thus selectively kill cancer cells and have a lower level of toxicity on normal tissues than traditional chemotherapeutics.
Although identifying the synthetic lethal pathway with certain DNA repair defects of cancer cells is an established concept (Hartwell et al. 1997), recent progress in reverse genetic screening using CRISPR/Cas9 technology and novel targeting strategies such as proteolysis targeting chimeras (PROTACs) (Schapira et al. 2019) has opened up many new opportunities in this area. An excellent example of the synthetic lethal interaction is the synergistic effect observed between the BRCA1/BRCA2 and PARP1 pathways. PARP1 inhibition selectively leads to cell death in BRCA1- or BRCA2-deficient cancer cells (Bryant et al. 2005; Farmer et al. 2005): this finding has been validated in a mouse model (Farmer et al. 2005) and in clinical settings (Ashworth and Lord 2018). Similarly, synthetic lethal interactions between MSH2 and POLB, MLH1 and POLG, RAD54B and FEN1, and MLH1/MSH2 and PTEN-induced putative kinase 1 genes have been suggested (Martin et al. 2010; van Pel et al. 2013; McManus et al. 2009). More recently, interactions between FEN1, APEX2 and BRCA2, between RNASEH2B and PAPR1, and between PolQ and PARP1 have been identified (Mengwasser et al. 2019; Zimmermann et al. 2018; Ceccaldi et al. 2015). Using CRISPR/Cas9 screening, Behan et al. prioritized therapeutic targets in different cancer types, and discovered additional synthetic lethal interactions (Behan et al. 2019). Furthermore, methotrexate might have a preferential effect on MMR-defective cancer cells (Martin et al. 2009), and its combination with PARP inhibitors could be particularly effective against MMR-deficient cancers due to synthetic lethal interactions (McCabe et al. 2006; Vilar et al. 2011; Miquel et al. 2007).
New therapeutic attempts are also being explored to target specific features of genomic instability, such as centrosome amplification induced by TP53 loss or 5- fluorouracil treatment that can promote chromosomal instability (Lee et al. 2010). As supernumerary centrosomes are problematic for cancer cells to divide, the centrosome clustering pathway might offer a vulnerability for therapeutic intervention (Kramer et al. 2011). Indeed, Griseofulvin was shown to target microtubule dynamics aspects of the centrosome clustering pathway and to de-cluster centrosome to selectively inhibit cancer cell proliferation (Ogden et al. 2012). Similarly, kinesin-like motor protein silencing can inhibit the centrosome clustering pathway and kill cancer cells with supernumerary centrosomes (Kwon et al. 2008).
Key DDR transducers include ATM, ATR, CHK1 and CHK2, and their inhibitors recently showed promise in clinical settings when combined with chemotherapeutics (Roos et al. 2016). In addition, the translesion repair enzyme (REV1) small molecule inhibitor JH-RE-06 sensitizes tumors to cisplatin and reduces cisplatin-induced mutagenesis (Wojtaszek et al. 2019).
Many traditional chemotherapeutics already exploit the genomic instability of cancer cells. However, there are emerging new targeted strategies against different features of genomic instability, providing potentially new and improved treatments to try in clinical settings.
Future perspectives
During cancer evolution, cell plasticity and genomic instability can be affected by both cancer genes (including oncogenes and tumor suppressors) and therapeutic drugs. While the interaction between these two kinds of drivers might have a critical role in both treatment-induced mutagenesis and selection pressure, explorations in this area are sparse. Recently, it was revealed that the TMZ mutational signature can only be generated in MMR-deficient gliomas (Touat et al. 2020). Studying the interaction between therapeutic agents and the genetic background here might yield insights into the clonal evolution mechanism, the optimal usage of therapeutic drugs and new therapeutic strategy.
Although genomic instability of subclones could promote their adaptability and acquisition of resistance mutations, they need to overcome therapy-induced or oncogenic stress-induced senescence and apoptosis (Di Micco et al. 2006; Bartkova et al. 2006) to become the dominant relapse clones (Fig. 4). To resolve the contradiction between plasticity and proliferation, cancer cells need to balance genomic instability and rapid expansion during evolution (Bulock et al. 2020), though limited experimental evidence for this occurring is available. One possible mechanism is to lose wild-type p53 to suppress senescence and apoptosis (Halazonetis et al. 2008), another is to temporally regulate the genetic instability of cancer cells (unpublished data), but the mechanisms of these approaches remain to be fully elucidated. The results of such mechanistic studies, however, could help identify new therapeutic strategies for disease prevention and intervention.
Fig. 4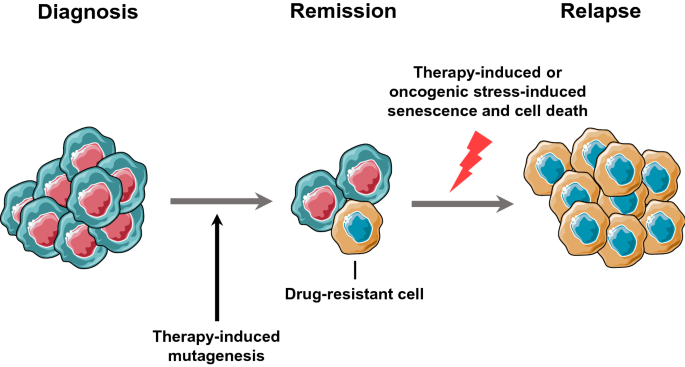
Therapy has a double-edged sword effect during cancer evolution. Cancer therapy has the potential to cause genetic instability and mutagenesis, which promotes the emergence of drug-resistant subclones. However, therapy can also induce cancer cell senescence and death, which would be a barrier to clonal expansion of resistant subclones
Full size imageReferences
Alexandrov, L. B., Ju, Y. S., Haase, K., Van Loo, P., Martincorena, I., Nik-Zainal, S., et al. (2016). Mutational signatures associated with tobacco smoking in human cancer. Science, 354(6312), 618–622. https://doi.org/10.1126/science.aag0299.
Alexandrov, L. B., Kim, J., Haradhvala, N. J., Huang, M. N., Tian Ng, A. W., Wu, Y., et al. (2020). The repertoire of mutational signatures in human cancer. Nature, 578(7793), 94–101. https://doi.org/10.1038/s41586-020-1943-3.
Alexandrov, L. B., Nik-Zainal, S., Wedge, D. C., Aparicio, S. A., Behjati, S., Biankin, A. V., et al. (2013). Signatures of mutational processes in human cancer. Nature, 500(7463), 415–421. https://doi.org/10.1038/nature12477.
Ashworth, A., & Lord, C. J. (2018). Synthetic lethal therapies for cancer: What's next after PARP inhibitors? Nature Reviews Clinical Oncology, 15(9), 564–576. https://doi.org/10.1038/s41571-018-0055-6.
Bartkova, J., Rezaei, N., Liontos, M., Karakaidos, P., Kletsas, D., Issaeva, N., et al. (2006). Oncogene-induced senescence is part of the tumorigenesis barrier imposed by DNA damage checkpoints. Nature, 444(7119), 633–637. https://doi.org/10.1038/nature05268.
Bean, J., Brennan, C., Shih, J. Y., Riely, G., Viale, A., Wang, L., et al. (2007). MET amplification occurs with or without T790M mutations in EGFR mutant lung tumors with acquired resistance to gefitinib or erlotinib. Proceedings of the National Academy of Sciences, 104(52), 20932–20937. https://doi.org/10.1073/pnas.0710370104.
Behan, F. M., Iorio, F., Picco, G., Goncalves, E., Beaver, C. M., Migliardi, G., et al. (2019). Prioritization of cancer therapeutic targets using CRISPR-Cas9 screens. Nature, 568(7753), 511–516. https://doi.org/10.1038/s41586-019-1103-9.
Bian, L., Meng, Y., Zhang, M., & Li, D. (2019). MRE11-RAD50-NBS1 complex alterations and DNA damage response: Implications for cancer treatment. Molecular Cancer, 18(1), 169. https://doi.org/10.1186/s12943-019-1100-5.
Boot, A., Huang, M. N., Ng, A. W. T., Ho, S. C., Lim, J. Q., Kawakami, Y., et al. (2018). In-depth characterization of the cisplatin mutational signature in human cell lines and in esophageal and liver tumors. Genome Research, 28(5), 654–665. https://doi.org/10.1101/gr.230219.117.
Boumahdi, S., & de Sauvage, F. J. (2020). The great escape: Tumour cell plasticity in resistance to targeted therapy. Nature Reviews Drug Discovery, 19(1), 39–56. https://doi.org/10.1038/s41573-019-0044-1.
Bryant, H. E., Schultz, N., Thomas, H. D., Parker, K. M., Flower, D., Lopez, E., et al. (2005). Specific killing of BRCA2-deficient tumours with inhibitors of poly(ADP-ribose) polymerase. Nature, 434(7035), 913–917. https://doi.org/10.1038/nature03443.
Bulock, C. R., Xing, X., & Shcherbakova, P. V. (2020). Mismatch repair and DNA polymerase delta proofreading prevent catastrophic accumulation of leading strand errors in cells expressing a cancer-associated DNA polymerase variant. Nucleic Acids Research. https://doi.org/10.1093/nar/gkaa633.
Burns, M. B., Lackey, L., Carpenter, M. A., Rathore, A., Land, A. M., Leonard, B., et al. (2013). APOBEC3B is an enzymatic source of mutation in breast cancer. Nature, 494(7437), 366–370. https://doi.org/10.1038/nature11881.
Cahill, D. P., Lengauer, C., Yu, J., Riggins, G. J., Willson, J. K., Markowitz, S. D., et al. (1998). Mutations of mitotic checkpoint genes in human cancers. Nature, 392(6673), 300–303. https://doi.org/10.1038/32688.
Campbell, B. B., Light, N., Fabrizio, D., Zatzman, M., Fuligni, F., de Borja, R., et al. (2017). Comprehensive analysis of hypermutation in human cancer. Cell, 171(5), 1042–1056. https://doi.org/10.1016/j.cell.2017.09.048.
Canela, A., Sridharan, S., Sciascia, N., Tubbs, A., Meltzer, P., Sleckman, B. P., et al. (2016). DNA breaks and end resection measured genome-wide by end sequencing. Molecular Cell, 63(5), 898–911. https://doi.org/10.1016/j.molcel.2016.06.034.
Ceccaldi, R., Liu, J. C., Amunugama, R., Hajdu, I., Primack, B., Petalcorin, M. I., et al. (2015). Homologous-recombination-deficient tumours are dependent on Poltheta-mediated repair. Nature, 518(7538), 258–262. https://doi.org/10.1038/nature14184.
Chae, Y. K., Davis, A. A., Raparia, K., Agte, S., Pan, A., Mohindra, N., et al. (2019). Association of tumor mutational burden with DNA repair mutations and response to anti-PD-1/PD-L1 therapy in non-small-cell lung cancer. Clinical Lung Cancer, 20(2), 88–96. https://doi.org/10.1016/j.cllc.2018.09.008.
Cheng, C., Zhou, Y., Li, H., Xiong, T., Li, S., Bi, Y., et al. (2016). Whole-genome sequencing reveals diverse models of structural variations in esophageal squamous cell carcinoma. American Journal of Human Genetics, 98(2), 256–274. https://doi.org/10.1016/j.ajhg.2015.12.013.
Crosetto, N., Mitra, A., Silva, M. J., Bienko, M., Dojer, N., Wang, Q., et al. (2013). Nucleotide-resolution DNA double-strand break mapping by next-generation sequencing. Nature Methods, 10(4), 361–365. https://doi.org/10.1038/nmeth.2408.
Di Micco, R., Fumagalli, M., Cicalese, A., Piccinin, S., Gasparini, P., Luise, C., et al. (2006). Oncogene-induced senescence is a DNA damage response triggered by DNA hyper-replication. Nature, 444(7119), 638–642. https://doi.org/10.1038/nature05327.
Diaz, L. A., Jr., Williams, R. T., Wu, J., Kinde, I., Hecht, J. R., Berlin, J., et al. (2012). The molecular evolution of acquired resistance to targeted EGFR blockade in colorectal cancers. Nature, 486(7404), 537–540. https://doi.org/10.1038/nature11219.
Farmer, H., McCabe, N., Lord, C. J., Tutt, A. N., Johnson, D. A., Richardson, T. B., et al. (2005). Targeting the DNA repair defect in BRCA mutant cells as a therapeutic strategy. Nature, 434(7035), 917–921. https://doi.org/10.1038/nature03445.
Felix, C. A., Hosler, M. R., Winick, N. J., Masterson, M., Wilson, A. E., & Lange, B. J. (1995). ALL-1 gene rearrangements in DNA topoisomerase II inhibitor-related leukemia in children. Blood, 85(11), 3250–3256.
Ferrando, A. A., & Lopez-Otin, C. (2017). Clonal evolution in leukemia. Nature Medicine, 23(10), 1135–1145. https://doi.org/10.1038/nm.4410.
Garcia-Muse, T., & Aguilera, A. (2016). Transcription-replication conflicts: How they occur and how they are resolved. Nature Reviews Molecular Cell Biology, 17(9), 553–563. https://doi.org/10.1038/nrm.2016.88.
Goodfellow, P. J., Buttin, B. M., Herzog, T. J., Rader, J. S., Gibb, R. K., Swisher, E., et al. (2003). Prevalence of defective DNA mismatch repair and MSH6 mutation in an unselected series of endometrial cancers. Proceedings of the National Academy of Sciences, 100(10), 5908–5913. https://doi.org/10.1073/pnas.1030231100.
Halazonetis, T. D., Gorgoulis, V. G., & Bartek, J. (2008). An oncogene-induced DNA damage model for cancer development. Science, 319(5868), 1352–1355. https://doi.org/10.1126/science.1140735.
Hanahan, D., & Weinberg, R. A. (2011). Hallmarks of cancer: The next generation. Cell, 144(5), 646–674. https://doi.org/10.1016/j.cell.2011.02.013.
Hartwell, L. H., Szankasi, P., Roberts, C. J., Murray, A. W., & Friend, S. H. (1997). Integrating genetic approaches into the discovery of anticancer drugs. Science, 278(5340), 1064–1068. https://doi.org/10.1126/science.278.5340.1064.
Hu, Z. I., Shia, J., Stadler, Z. K., Varghese, A. M., Capanu, M., Salo-Mullen, E., et al. (2018). Evaluating mismatch repair deficiency in pancreatic adenocarcinoma: Challenges and recommendations. Clinical Cancer Research, 24(6), 1326–1336. https://doi.org/10.1158/1078-0432.CCR-17-3099.
Ibrahim, Y. H., Garcia-Garcia, C., Serra, V., He, L., Torres-Lockhart, K., Prat, A., et al. (2012). PI3K inhibition impairs BRCA1/2 expression and sensitizes BRCA-proficient triple-negative breast cancer to PARP inhibition. Cancer Discovery, 2(11), 1036–1047. https://doi.org/10.1158/2159-8290.CD-11-0348.
Jeggo, P. A., Pearl, L. H., & Carr, A. M. (2016). DNA repair, genome stability and cancer: A historical perspective. Nature Reviews Cancer, 16(1), 35–42. https://doi.org/10.1038/nrc.2015.4.
Juvekar, A., Burga, L. N., Hu, H., Lunsford, E. P., Ibrahim, Y. H., Balmana, J., et al. (2012). Combining a PI3K inhibitor with a PARP inhibitor provides an effective therapy for BRCA1-related breast cancer. Cancer Discovery, 2(11), 1048–1063. https://doi.org/10.1158/2159-8290.CD-11-0336.
Kirstein, A., Schmid, T. E., & Combs, S. E. (2020). The role of miRNA for the treatment of MGMT unmethylated glioblastoma multiforme. Cancers (Basel). https://doi.org/10.3390/cancers12051099.
Kong, X., Kuilman, T., Shahrabi, A., Boshuizen, J., Kemper, K., Song, J. Y., et al. (2017). Cancer drug addiction is relayed by an ERK2-dependent phenotype switch. Nature, 550(7675), 270–274. https://doi.org/10.1038/nature24037.
Kramer, A., Maier, B., & Bartek, J. (2011). Centrosome clustering and chromosomal (in)stability: A matter of life and death. Molecular Oncology, 5(4), 324–335. https://doi.org/10.1016/j.molonc.2011.05.003.
Kucab, J. E., Zou, X., Morganella, S., Joel, M., Nanda, A. S., Nagy, E., et al. (2019). A compendium of mutational signatures of environmental agents. Cell, 177(4), 821–836. https://doi.org/10.1016/j.cell.2019.03.001.
Kwon, M., Godinho, S. A., Chandhok, N. S., Ganem, N. J., Azioune, A., Thery, M., et al. (2008). Mechanisms to suppress multipolar divisions in cancer cells with extra centrosomes. Genes and Development, 22(16), 2189–2203. https://doi.org/10.1101/gad.1700908.
Lee, S. C., Chan, J. Y., & Pervaiz, S. (2010). Spontaneous and 5-fluorouracil-induced centrosome amplification lowers the threshold to resveratrol-evoked apoptosis in colon cancer cells. Cancer Letters, 288(1), 36–41. https://doi.org/10.1016/j.canlet.2009.06.020.
Li, B., Brady, S. W., Ma, X., Shen, S., Zhang, Y., Li, Y., et al. (2020). Therapy-induced mutations drive the genomic landscape of relapsed acute lymphoblastic leukemia. Blood, 135(1), 41–55. https://doi.org/10.1182/blood.2019002220.
Li, D., Liu, H., Jiao, L., Chang, D. Z., Beinart, G., Wolff, R. A., et al. (2006). Significant effect of homologous recombination DNA repair gene polymorphisms on pancreatic cancer survival. Cancer Research, 66(6), 3323–3330. https://doi.org/10.1158/0008-5472.CAN-05-3032.
Lipinski, K. A., Barber, L. J., Davies, M. N., Ashenden, M., Sottoriva, A., & Gerlinger, M. (2016). Cancer evolution and the limits of predictability in precision cancer medicine. Trends Cancer, 2(1), 49–63. https://doi.org/10.1016/j.trecan.2015.11.003.
Macheret, M., & Halazonetis, T. D. (2018). Intragenic origins due to short G1 phases underlie oncogene-induced DNA replication stress. Nature, 555(7694), 112–116. https://doi.org/10.1038/nature25507.
Martin, S. A., McCabe, N., Mullarkey, M., Cummins, R., Burgess, D. J., Nakabeppu, Y., et al. (2010). DNA polymerases as potential therapeutic targets for cancers deficient in the DNA mismatch repair proteins MSH2 or MLH1. Cancer Cell, 17(3), 235–248. https://doi.org/10.1016/j.ccr.2009.12.046.
Martin, S. A., McCarthy, A., Barber, L. J., Burgess, D. J., Parry, S., Lord, C. J., et al. (2009). Methotrexate induces oxidative DNA damage and is selectively lethal to tumour cells with defects in the DNA mismatch repair gene MSH2. EMBO Molecular Medicine, 1(6–7), 323–337. https://doi.org/10.1002/emmm.200900040.
Martincorena, I., Roshan, A., Gerstung, M., Ellis, P., Van Loo, P., McLaren, S., et al. (2015). Tumor evolution: High burden and pervasive positive selection of somatic mutations in normal human skin. Science, 348(6237), 880–886. https://doi.org/10.1126/science.aaa6806.
Matsumoto, Y., Marusawa, H., Kinoshita, K., Endo, Y., Kou, T., Morisawa, T., et al. (2007). Helicobacter pylori infection triggers aberrant expression of activation-induced cytidine deaminase in gastric epithelium. Nature Medicine, 13(4), 470–476. https://doi.org/10.1038/nm1566.
McCabe, N., Turner, N. C., Lord, C. J., Kluzek, K., Bialkowska, A., Swift, S., et al. (2006). Deficiency in the repair of DNA damage by homologous recombination and sensitivity to poly(ADP-ribose) polymerase inhibition. Cancer Research, 66(16), 8109–8115. https://doi.org/10.1158/0008-5472.CAN-06-0140.
McKenzie, G. J., & Rosenberg, S. M. (2001). Adaptive mutations, mutator DNA polymerases and genetic change strategies of pathogens. Current Opinion in Microbiology, 4(5), 586–594. https://doi.org/10.1016/s1369-5274(00)00255-1.
McManus, K. J., Barrett, I. J., Nouhi, Y., & Hieter, P. (2009). Specific synthetic lethal killing of RAD54B-deficient human colorectal cancer cells by FEN1 silencing. Proceedings of the National Academy of Sciences, 106(9), 3276–3281. https://doi.org/10.1073/pnas.0813414106.
Mengwasser, K. E., Adeyemi, R. O., Leng, Y., Choi, M. Y., Clairmont, C., D'Andrea, A. D., et al. (2019). Genetic screens reveal FEN1 and APEX2 as BRCA2 synthetic lethal targets. Molecular Cell, 73(5), 885–899. https://doi.org/10.1016/j.molcel.2018.12.008.
Miquel, C., Jacob, S., Grandjouan, S., Aime, A., Viguier, J., Sabourin, J. C., et al. (2007). Frequent alteration of DNA damage signalling and repair pathways in human colorectal cancers with microsatellite instability. Oncogene, 26(40), 5919–5926. https://doi.org/10.1038/sj.onc.1210419.
Misale, S., Yaeger, R., Hobor, S., Scala, E., Janakiraman, M., Liska, D., et al. (2012). Emergence of KRAS mutations and acquired resistance to anti-EGFR therapy in colorectal cancer. Nature, 486(7404), 532–536. https://doi.org/10.1038/nature11156.
Morganella, S., Alexandrov, L. B., Glodzik, D., Zou, X., Davies, H., Staaf, J., et al. (2016). The topography of mutational processes in breast cancer genomes. Nature Communication, 7, 11383. https://doi.org/10.1038/ncomms11383.
Mouw, K. W., Goldberg, M. S., Konstantinopoulos, P. A., & D'Andrea, A. D. (2017). DNA damage and repair biomarkers of immunotherapy response. Cancer Discovery, 7(7), 675–693. https://doi.org/10.1158/2159-8290.CD-17-0226.
Nik-Zainal, S., Davies, H., Staaf, J., Ramakrishna, M., Glodzik, D., Zou, X., et al. (2016). Landscape of somatic mutations in 560 breast cancer whole-genome sequences. Nature, 534(7605), 47–54. https://doi.org/10.1038/nature17676.
Nogales, V., Reinhold, W. C., Varma, S., Martinez-Cardus, A., Moutinho, C., Moran, S., et al. (2016). Epigenetic inactivation of the putative DNA/RNA helicase SLFN11 in human cancer confers resistance to platinum drugs. Oncotarget, 7(3), 3084–3097. https://doi.org/10.18632/oncotarget.6413.
Nowell, P. C. (1976). The clonal evolution of tumor cell populations. Science, 194(4260), 23–28. https://doi.org/10.1126/science.959840.
Ogden, A., Rida, P. C., & Aneja, R. (2012). Let's huddle to prevent a muddle: Centrosome declustering as an attractive anticancer strategy. Cell Death and Differentiation, 19(8), 1255–1267. https://doi.org/10.1038/cdd.2012.61.
Okazaki, S., Shintani, S., Hirata, Y., Suina, K., Semba, T., Yamasaki, J., et al. (2018). Synthetic lethality of the ALDH3A1 inhibitor dyclonine and xCT inhibitors in glutathione deficiency-resistant cancer cells. Oncotarget, 9(73), 33832–33843. https://doi.org/10.18632/oncotarget.26112.
Panchakshari, R. A., Zhang, X., Kumar, V., Du, Z., Wei, P. C., Kao, J., et al. (2018). DNA double-strand break response factors influence end-joining features of IgH class switch and general translocation junctions. Proceedings of the National Academy of Sciences, 115(4), 762–767. https://doi.org/10.1073/pnas.1719988115.
Rizvi, N. A., Hellmann, M. D., Snyder, A., Kvistborg, P., Makarov, V., Havel, J. J., et al. (2015). Cancer immunology. Mutational landscape determines sensitivity to PD-1 blockade in non-small cell lung cancer. Science, 348(6230), 124–128. https://doi.org/10.1126/science.aaa1348.
Roos, W. P., Thomas, A. D., & Kaina, B. (2016). DNA damage and the balance between survival and death in cancer biology. Nature Reviews Cancer, 16(1), 20–33. https://doi.org/10.1038/nrc.2015.2.
Russo, M., Crisafulli, G., Sogari, A., Reilly, N. M., Arena, S., Lamba, S., et al. (2019). Adaptive mutability of colorectal cancers in response to targeted therapies. Science, 366(6472), 1473–1480. https://doi.org/10.1126/science.aav4474.
Sawada, G., Niida, A., Uchi, R., Hirata, H., Shimamura, T., Suzuki, Y., et al. (2016). Genomic landscape of esophageal squamous cell carcinoma in a Japanese population. Gastroenterology, 150(5), 1171–1182. https://doi.org/10.1053/j.gastro.2016.01.035.
Schapira, M., Calabrese, M. F., Bullock, A. N., & Crews, C. M. (2019). Targeted protein degradation: Expanding the toolbox. Nature Reviews Drug Discovery, 18(12), 949–963. https://doi.org/10.1038/s41573-019-0047-y.
Sotillo, R., Schvartzman, J. M., Socci, N. D., & Benezra, R. (2010). Mad2-induced chromosome instability leads to lung tumour relapse after oncogene withdrawal. Nature, 464(7287), 436–440. https://doi.org/10.1038/nature08803.
Touat, M., Li, Y. Y., Boynton, A. N., Spurr, L. F., Iorgulescu, J. B., Bohrson, C. L., et al. (2020). Mechanisms and therapeutic implications of hypermutation in gliomas. Nature, 580(7804), 517–523. https://doi.org/10.1038/s41586-020-2209-9.
van Pel, D. M., Barrett, I. J., Shimizu, Y., Sajesh, B. V., Guppy, B. J., Pfeifer, T., et al. (2013). An evolutionarily conserved synthetic lethal interaction network identifies FEN1 as a broad-spectrum target for anticancer therapeutic development. PLoS Genetics, 9(1), e1003254. https://doi.org/10.1371/journal.pgen.1003254.
Venkitaraman, A. R. (2011). Does metabolite deficiency mark oncogenic cell cycles? Cell, 145(3), 337–338. https://doi.org/10.1016/j.cell.2011.04.003.
Vilar, E., Bartnik, C. M., Stenzel, S. L., Raskin, L., Ahn, J., Moreno, V., et al. (2011). MRE11 deficiency increases sensitivity to poly(ADP-ribose) polymerase inhibition in microsatellite unstable colorectal cancers. Cancer Research, 71(7), 2632–2642. https://doi.org/10.1158/0008-5472.CAN-10-1120.
Wheeler, J. M., Bodmer, W. F., & Mortensen, N. J. (2000). DNA mismatch repair genes and colorectal cancer. Gut, 47(1), 148–153. https://doi.org/10.1136/gut.47.1.148.
Wojtaszek, J. L., Chatterjee, N., Najeeb, J., Ramos, A., Lee, M., Bian, K., et al. (2019). A small molecule targeting mutagenic translesion synthesis improves chemotherapy. Cell, 178(1), 152–159. https://doi.org/10.1016/j.cell.2019.05.028.
Yokoyama, A., Kakiuchi, N., Yoshizato, T., Nannya, Y., Suzuki, H., Takeuchi, Y., et al. (2019). Age-related remodelling of oesophageal epithelia by mutated cancer drivers. Nature, 565(7739), 312–317. https://doi.org/10.1038/s41586-018-0811-x.
Yuan, S., Norgard, R. J., & Stanger, B. Z. (2019). Cellular plasticity in cancer. Cancer Discovery, 9(7), 837–851. https://doi.org/10.1158/2159-8290.CD-19-0015.
Zhou, B. B., & Elledge, S. J. (2000). The DNA damage response: Putting checkpoints in perspective. Nature, 408(6811), 433–439. https://doi.org/10.1038/35044005.
Zhou, B. B., Peyton, M., He, B., Liu, C., Girard, L., Caudler, E., et al. (2006). Targeting ADAM-mediated ligand cleavage to inhibit HER3 and EGFR pathways in non-small cell lung cancer. Cancer Cell, 10(1), 39–50. https://doi.org/10.1016/j.ccr.2006.05.024.
Zimmermann, M., Murina, O., Reijns, M. A. M., Agathanggelou, A., Challis, R., Tarnauskaite, Z., et al. (2018). CRISPR screens identify genomic ribonucleotides as a source of PARP-trapping lesions. Nature, 559(7713), 285–289. https://doi.org/10.1038/s41586-018-0291-z.
Zou, L., & Elledge, S. J. (2003). Sensing DNA damage through ATRIP recognition of RPA-ssDNA complexes. Science, 300(5625), 1542–1548. https://doi.org/10.1126/science.1083430.
Author information
Affiliations
Key Laboratory of Pediatric Hematology and Oncology Ministry of Health, Pediatric Translational Medicine Institute, Shanghai Children’s Medical Center, Shanghai Jiao Tong University School of Medicine, Shanghai, China
Yan Xu, Hui Li, Fan Yang, Dingpeng Yang & Bin-Bing S. Zhou
Department of Pharmacology and Chemical Biology, School of Basic Medicine and Collaborative Innovation Center for Translational Medicine, Shanghai Jiao Tong University School of Medicine, Shanghai, China
Hui Li & Bin-Bing S. Zhou
Corresponding author
Correspondence to Bin-Bing S. Zhou.
Rights and permissions
About this article
Cite this article
Xu, Y., Li, H., Yang, F. et al. Cell plasticity and genomic instability in cancer evolution. GENOME INSTAB. DIS. (2020). https://doi.org/10.1007/s42764-020-00023-w
Received30 May 2020
Revised26 August 2020
Accepted15 September 2020
Published06 October 2020
Share this article
Anyone you share the following link with will be able to read this content:
Get shareable linkKeywords
Oncogene
Tumor suppressor
Cell plasticity
Genomic instability
Cancer evolution
用户登录
还没有账号?
立即注册